Image above generated by AI for this analysis (Imagen 3-002)1Image generated in a few seconds, on 22 December 2024, via Imagen 3-002, text prompt by Alan D. Thompson, ‘a zoomed out background header for ozone in the stratosphere, with lowercase title ‘o3’, otherworldly colors.’
Advising organizations from Apple to the US Gov, & cited in the new G7 AI doc.†Get The Memo.
Alan D. Thompson
December 2024
Summary
Organization | OpenAI |
Model name | o3 (OpenAI model number three) |
Internal/project name | – |
Model type | Multimodal |
Parameter count | See below: Size estimate |
Dataset size (tokens) | See report: What’s in GPT-5? |
Training data end date | Jun/2024 (est) |
Training start date | Sep/2024 (est) |
Training end/convergence date | Nov/2024 (est) |
Training time (total) | –![]() |
Announce date | 20/Dec/2024 |
Release date (public) | o3-mini: 31/Jan/2025 o3: 2025Q1 |
Paper | o3-mini-system-card (PDF) |
Playground | – |
Updates
31/Jan/2025: o3-mini released, GPQA=77.0, GPQA=79.7 for ‘high’ thinking. ALPrompt 2025H1=1/5. My analysis is that this model’s performance is very poor, with responses often becoming disordered and illogical. OpenAI compared o3-mini to OpenAI’s software engineers, and it performed very poorly (o3-mini=0%, o1=12%).
o3-mini models have the lowest performance, with scores of 0%… We suspect o3-mini’s low performance is due to poor instruction following and confusion about specifying tools in the correct format. The model often attempts to use a hallucinated bash tool rather than python despite constant, multi-shot prompting and feedback that this format is incorrect. This resulted in long conversations that likely hurt its performance. (o3-mini paper, p31)
23/Jan/2025: Brad Lightcap, OpenAI (Cisco AI Summit, 23/Jan/2025)
The great insight that Ilya had way back when is that the systems that didn’t seem to work at small scale weren’t fundamentally flawed, they just needed to be scaled up. And we’ve seen demonstrably now that when you scale GPT models, you get consistently better results. We think that continues. Now you have this entirely new vector of scaling, which is the o series of models. We’re very excited about that, and we don’t see any asymptote on scaling laws…
With scaling the big pre-trained transformers, you enter into a regime where you become data limited if you’re not already compute limited. And so in some sense, we hit a wall. It wasn’t a technical wall, it was just an environmental wall. So we could continue to scale those models. There’s no scientific reason why a GPT-5 or 6 couldn’t exist and have the same kind of return to scale that you see going from GPT-3 to GPT-4…
The beautiful thing about RL [reinforcement learning], though, and the way that we’ve built the reasoning models, is they take you on a different path. Someone on our research team described it to me the other day. o1 is almost like a portal to GPT-7 or GPT-8. It’s a way to leverage the knowledge in the pre-trained model, which is a GPT-4 scale model, in a way that basically gives you the effective compute of what a GPT-7 or GPT-8 would otherwise use, to achieve that same level of intelligence. It’s a pure discontinuity on the scaling…
I think we’ll continue to do both [GPT and o models]. I think we still see a lot of potential in pre-training levels, where you’ll see a 5 and a 6 and a 7 coming on that front as well. I think we’ll see where that all goes. Believe it or not, it’s actually almost more continuous than these discrete jumps now.
22/Jan/2025: OpenAI Chief Product Officer Kevin Weil said… ‘OpenAI expects to release its smarter GPT o3 model in February or March [2025].’ (WSJ, 22/Jan/2025)
17/Jan/2025: OpenAI CEO on the upcoming o3-mini model: ‘[o3-mini is] worse than o1 pro at most things (but FAST)… o3 is much smarter; we are turning our attention to that now. (and o3 pro?! [is mind-blowing])’ (Twitter, 17/Jan/2025)
20/Dec/2024: o3 benchmarks:
GPQA Diamond=87.7% (o1=78.3%)
AIME 2024 = 96.7% (only one question wrong)
Codeforces: 99.8th percentile (score = 2727, o1=P94/1891)
SWE-bench verified = 71.7% (o1=48.9%)
FrontierMath = 25.2% (o1=2%)
Fields Medalist Timothy Gowers on the hundreds of questions in the FrontierMath benchmark (Nov/2024):
‘…all looked like things I had no idea how to solve… Getting even one question right would be well beyond what we can do now, let alone saturating them.’ [To score 25.2%, o3 must have got at least 63 of 250 questions correct]
19/Dec/2024: o2 renamed to o3:
“OpenAI is currently prepping the next generation of its o1 reasoning model, which takes more time to “think” about questions users give it before responding, according to two people with knowledge of the effort.
However, due to a potential copyright or trademark conflict with O2, a British telecommunications service provider, OpenAI has considered calling the next update “o3” and skipping “o2,” these people said.”
— via theinformation.com
Sidenote: I am seriously disappointed that my super cool analysis title ‘o2: The breath of life’ will go to waste because of a phone company. :-(
3/Nov/2024: OpenAI’s first mention of o2 by OpenAI CEO: ‘I heard o2 gets 105% on GPQA’ (Twitter, 3/Nov/2024)
Major points
Model name
OpenAI (17/Oct/2024 timecode 21m11s, transcribed by Whisper):
We plan to continue developing and releasing models in the new OpenAI o1 series, as well as our GPT series. In addition to model updates, we expect to add web browsing, file and image uploading, and other features to [o1 to] make them more useful in use cases in ChatGPT. And while even today you are able to switch between models in the same conversation, like you saw in the demo, we’re working to enable ChatGPT to automatically choose the right model for your given prompt.
Smarts
Reasoning model scores: o1, o3, o4
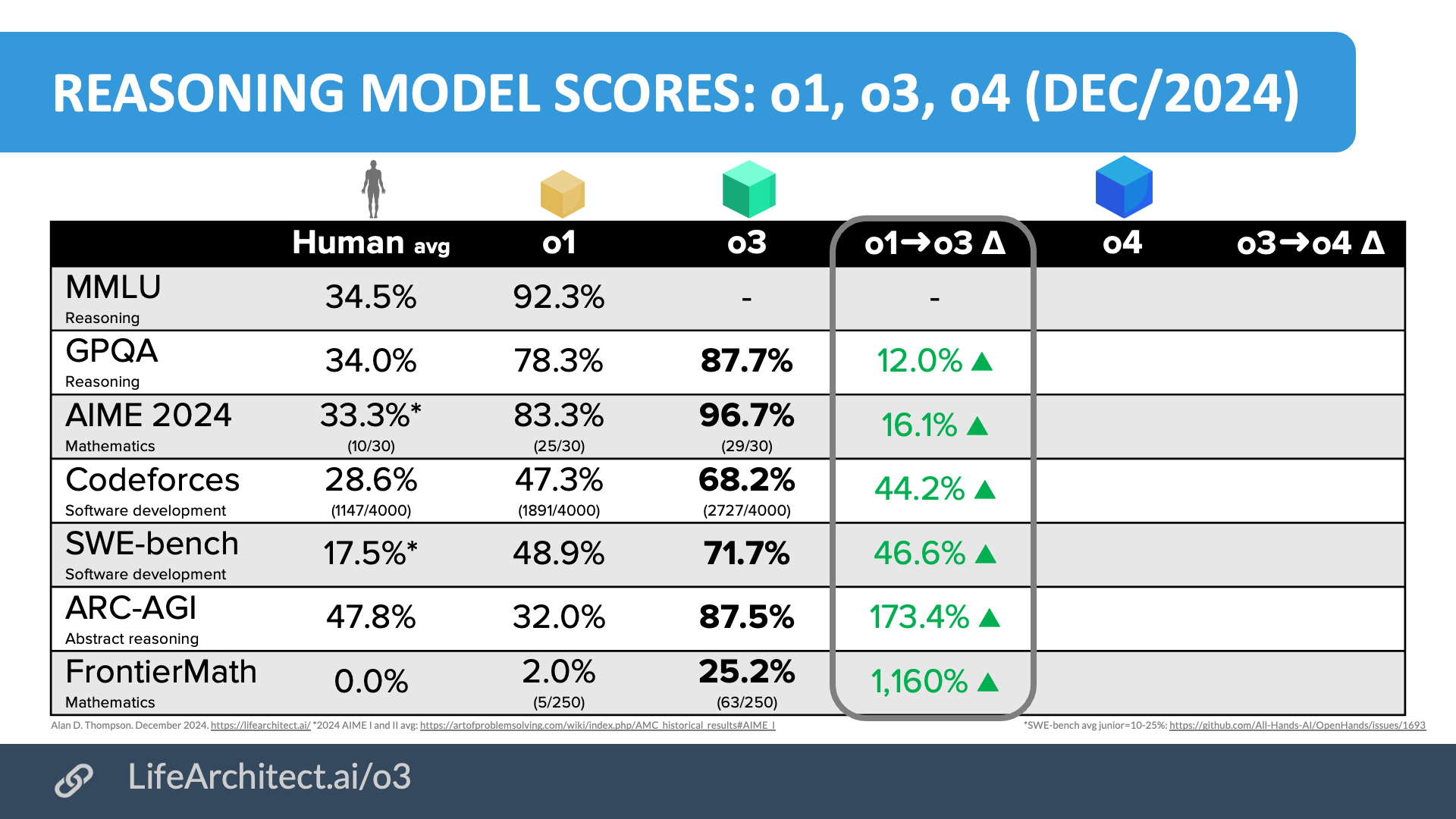
Human avg | o1 | o3 | o1→o3 Δ | o4 | o3→o4 Δ | |
---|---|---|---|---|---|---|
MMLU Reasoning |
34.5%2Human average, not expert average |
92.3% |
- | - | ||
GPQA Reasoning |
34.0%3PhD human average, but not PhD in subject field average |
78.3% |
87.7% |
12.0% ▲ |
||
AIME 2024 Mathematics |
33.3%(10/30)4"2024 AIME I and II median score=5/15": artofproblemsolving.com, disregards that these humans are probably in the top 1% of mathematics performance: 'Qualification parameters for the AIME depend on the results of the AMC 10 and AMC 12 competitions. For the AMC 10, at least the top 2.5% of all scorers from the A and B competition dates are invited, and for the AMC 12, at least the top 5% of all scorers on each version are invited [to take the AIME test].maa.org |
83.3%(25/30) |
96.7%(29/30) |
16.1% ▲ |
||
Codeforces Software development |
28.6%(1147/4000)5https://codeforces.com/blog/entry/126802 |
47.3%(1891/4000) |
68.2%(2727/4000) |
44.2% ▲ |
||
SWE-bench Software development |
17.5%6Human expert average, that is junior developer estimate=10-25%: https://github.com/All-Hands-AI/OpenHands/issues/1693 |
48.9% |
71.7% |
46.6% ▲ |
||
ARC-AGI Abstract reasoning |
47.8%7NYU: "Independent samples t-tests suggest that evaluation tasks are significantly harder for people than training tasks... We estimate that the average task accuracy after three attempts on the evaluation set is 64.2% (SD=22.8%, [55.9%, 68.9%]). In addition to this result, we report a first and second attempt average task accuracy of 47.8% (SD=23.2%, [41.6%, 54.6%] and 60.2% (SD=23.3%, [52.4%, 65.4%]) respectively." https://arxiv.org/pdf/2409.01374#page=6 |
32.0%8x.com |
87.5% |
173.4% ▲ |
||
FrontierMath Mathematics |
0.0%(0/250)9Human average estimate (by Alan) |
2.0%(5/250) |
25.2%(63/250) |
1,160% ▲ |
GPQA bubbles
See: AI + IQ testing (human vs AI)
Size estimate (o1)
Perhaps beginning with gpt-3.5-turbo (high performance with just 20B parameters, as revealed in a paper published and then quickly withdrawn by Microsoft in Oct/2023), the number of parameters in a model is no longer the primary indicator of the model’s power and capabilities. Other factors, such as architecture, training data quality, and inference optimization, now play equally important roles in determining a model’s overall performance.
In Apr/2021, Jones (now at Anthropic) released a paper called ‘Scaling Scaling Laws with Board Games’. It found that ‘for each additional 10× of train-time compute, about 15× of test-time compute can be eliminated.’
Reversing this relationship suggests that:
A 15× increase in inference-time compute would equate to a 10× increase in train-time compute.
Dr Noam Brown at OpenAI discussed this finding in a presentation to the Paul G. Allen School on 23/May/2024 and released to the public on 17/Sep/2024 (timecode is 28m17s).
In Aug/2024, Google DeepMind (with UC Berkeley) released a paper called ‘Scaling LLM Test-Time Compute Optimally can be More Effective than Scaling Model Parameters’. They found that:
“on problems where a smaller base model attains somewhat non-trivial success rates, test-time compute can be used to outperform a 14x larger model.
…
Test-time and pretraining compute are not 1-to-1 “exchangeable”. On easy and medium questions, which are within a model’s capabilities, or in settings with small inference requirement, test-time compute can easily cover up for additional pretraining. However, on challenging questions which are outside a given base model’s capabilities or under higher inference requirement, pretraining is likely more effective for improving performance.”
The table below is an extrapolation of DeepMind’s findings. It is oversimplified, all figures are rounded, and there may be a larger error margin as scale increases. I have added an ‘MoE equiv’ column, which shows the mixture-of-experts model size equivalent by applying a a 5× multiplier rule to the Dense model size (Standard inference-time compute, ITC).
Dense (Increased ITC) | Dense (Standard ITC) ×14 |
MoE equiv (Standard ITC) ×5 |
---|---|---|
1B | 14B | 70B |
7B | 98B | 490B |
8B | 112B | 560B |
20B | 280B | 1.4T |
25B | 350B | 1.76T (GPT-4) |
30B | 420B | 2.1T |
70B | 980B | 4.9T |
180B | 2.52T | 12.6T |
200B (o1) | 2.8T | 14T |
280B | 3.92T | 19.6T |
540B | 7.56T | 37.8T |
Read more at LifeArchitect.ai/o1
Dataset
A 200B parameter model trained on 20T tokens would have a tokens:parameters ratio of 100:1, an optimal pretraining ratio in 2024. See: Chinchilla data-optimal scaling laws: In plain English.
The o3 dataset is expected to use much of the initial GPT-3 dataset as detailed in my report What’s in my AI?, and to be very similar to the dataset used to train GPT-4 Classic 1.76T (available in lab Aug/2022), with some additional datasets from new synthetic data and partnerships as outlined in my GPT-5 dataset report.
A Comprehensive Analysis of Datasets Likely Used to Train GPT-5
Alan D. Thompson
LifeArchitect.ai
August 2024
27 pages incl title page, references, appendices.
Use cases
Coming soon…
2025 frontier AI models + highlights
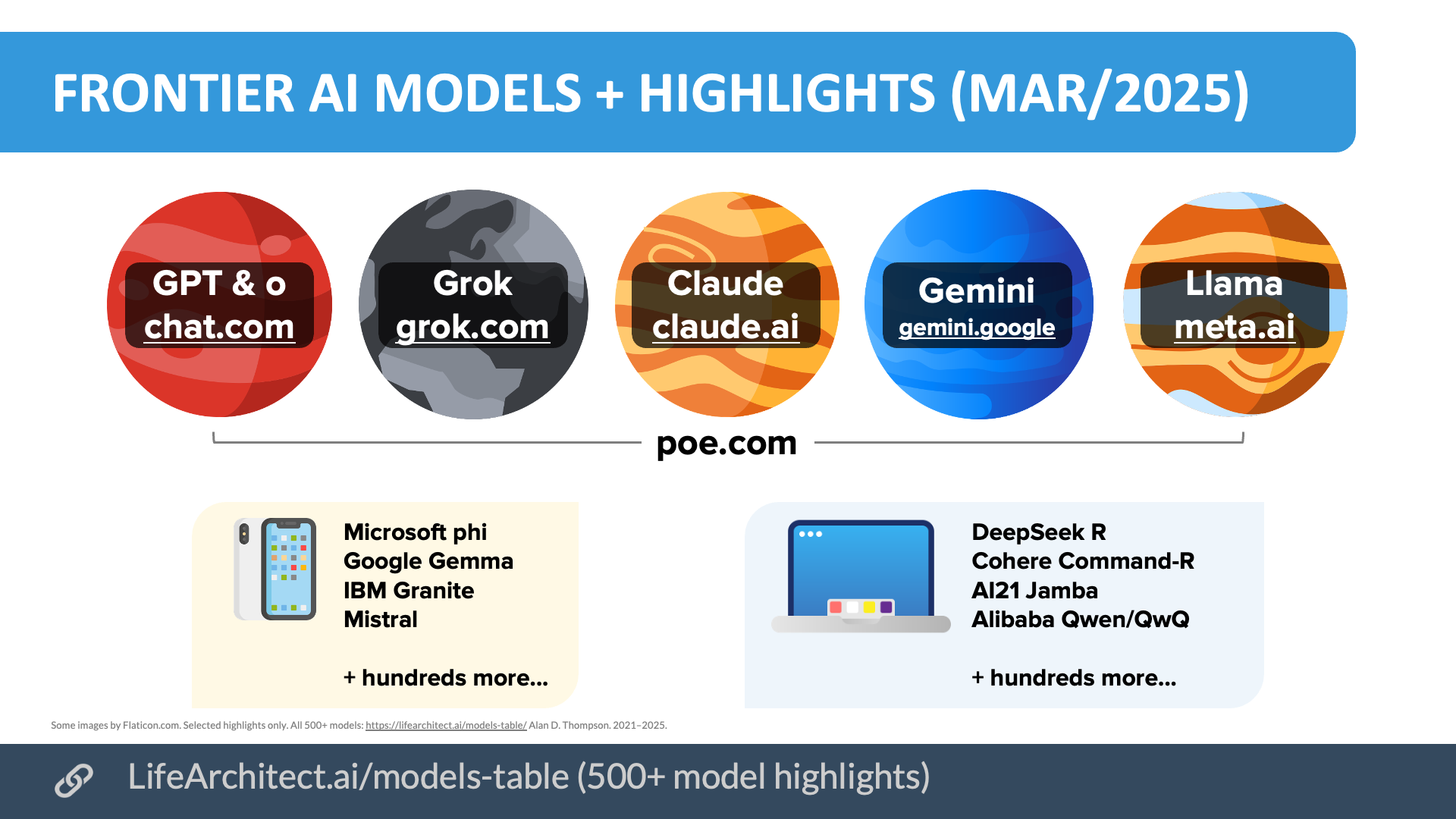
Permissions: Yes, you can use these visualizations anywhere, please leave the citation intact.
Models Table
Summary of current models: View the full data (Google sheets)Timeline to o3
Date | Milestone |
11/Jun/2018 | GPT-1 announced on the OpenAI blog. |
14/Feb/2019 | GPT-2 announced on the OpenAI blog. |
28/May/2020 | Initial GPT-3 preprint paper published to arXiv. |
11/Jun/2020 | GPT-3 API private beta. |
22/Sep/2020 | GPT-3 licensed to Microsoft. |
18/Nov/2021 | GPT-3 API opened to the public. |
27/Jan/2022 | InstructGPT released as text-davinci-002, now known as GPT-3.5. InstructGPT preprint paper Mar/2022. |
28/Jul/2022 | Exploring data-optimal models with FIM, paper on arXiv. |
1/Sep/2022 | GPT-3 model pricing cut by 66% for davinci model. |
21/Sep/2022 | Whisper (speech recognition) announced on the OpenAI blog. |
28/Nov/2022 | GPT-3.5 expanded to text-davinci-003, announced via email: 1. Higher quality writing. 2. Handles more complex instructions. 3. Better at longer form content generation. |
30/Nov/2022 | ChatGPT announced on the OpenAI blog. |
14/Mar/2023 | GPT-4 released. |
31/May/2023 | GPT-4 MathMix and step by step, paper on arXiv. |
6/Jul/2023 | GPT-4 available via API. |
25/Sep/2023 | GPT-4V finally released. |
13/May/2024 | GPT-4o announced. |
18/Jul/2024 | GPT-4o mini announced. |
12/Sep/2024 | o1 released. |
20/Dec/2024 | o3 announced. |
2025 | GPT-5… |
2025 | GPT-6… |
Videos
My livestream (link):
Interview (link):
OpenAI o3 evals (link):
Get The Memo
by Dr Alan D. Thompson · Be inside the lightning-fast AI revolution.Informs research at Apple, Google, Microsoft · Bestseller in 147 countries.
Artificial intelligence that matters, as it happens, in plain English.
Get The Memo.
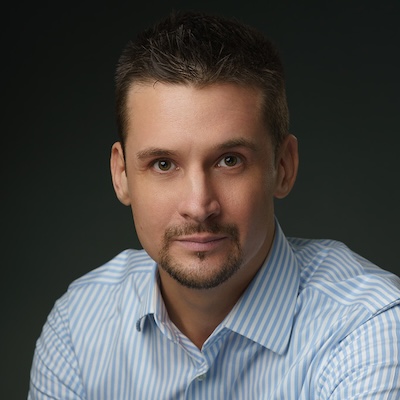
This page last updated: 1/Feb/2025. https://lifearchitect.ai/o3/↑
- 1Image generated in a few seconds, on 22 December 2024, via Imagen 3-002, text prompt by Alan D. Thompson, ‘a zoomed out background header for ozone in the stratosphere, with lowercase title ‘o3’, otherworldly colors.’
- 2Human average, not expert average
- 3PhD human average, but not PhD in subject field average
- 4"2024 AIME I and II median score=5/15": artofproblemsolving.com, disregards that these humans are probably in the top 1% of mathematics performance: 'Qualification parameters for the AIME depend on the results of the AMC 10 and AMC 12 competitions. For the AMC 10, at least the top 2.5% of all scorers from the A and B competition dates are invited, and for the AMC 12, at least the top 5% of all scorers on each version are invited [to take the AIME test].maa.org
- 5
- 6Human expert average, that is junior developer estimate=10-25%: https://github.com/All-Hands-AI/OpenHands/issues/1693
- 7NYU: "Independent samples t-tests suggest that evaluation tasks are significantly harder for people than training tasks... We estimate that the average task accuracy after three attempts on the evaluation set is 64.2% (SD=22.8%, [55.9%, 68.9%]). In addition to this result, we report a first and second attempt average task accuracy of 47.8% (SD=23.2%, [41.6%, 54.6%] and 60.2% (SD=23.3%, [52.4%, 65.4%]) respectively." https://arxiv.org/pdf/2409.01374#page=6
- 8
- 9Human average estimate (by Alan)