Get The Memo.
- Language model sizes
- Summary of current models
- Compute
- Context windows
- Achievements unlocked: Emergent abilities of LLMs
- Large language models: API or on-premise
- Increasing dataset sizes 2018-2023
- GPT-3’s top 10 datasets by domain/source
- Contents of GPT-3 & the Pile v1
- Contents of Chinese models
- Language model sizes & predictions
- Facebook BlenderBot 2.0 datasets by domain/source
- Facts on GPT-3
- Jurassic-1 by Israel’s AI21
- M6 by Alibaba
- BLOOM by BigScience
- Megatron by Google, NVIDIA, Facebook AI/UW, and NVIDIA/Microsoft
- InstructGPT by OpenAI one-pager
- WebGPT by OpenAI sample questions
- PaLM by Google: Explaining jokes + Inference chaining
- Luminous by Aleph Alpha
- DeepMind models (Gopher, Chinchilla, Flamingo, Gato)
- Google Imagen
- BriVL by RUC, China
- Perceiver by DeepMind
- AlexaTM 20B by Amazon Alexa AI
- DeepL using Attention vs Transformer
- Code Generation models
- Data/compute-optimal (Chinchilla)
- GPT-3.5 + ChatGPT
- 2022 model count
- Baidu ERNIE 3.0 Titan 260B (Wenxin)
- Together AI’s RedPajama dataset
- Imitation models & synthetic data; from Alpaca to Phoenix
- Tiny models + Apple UniLM 34M
Language model sizes
2024 optimal LLM highlights
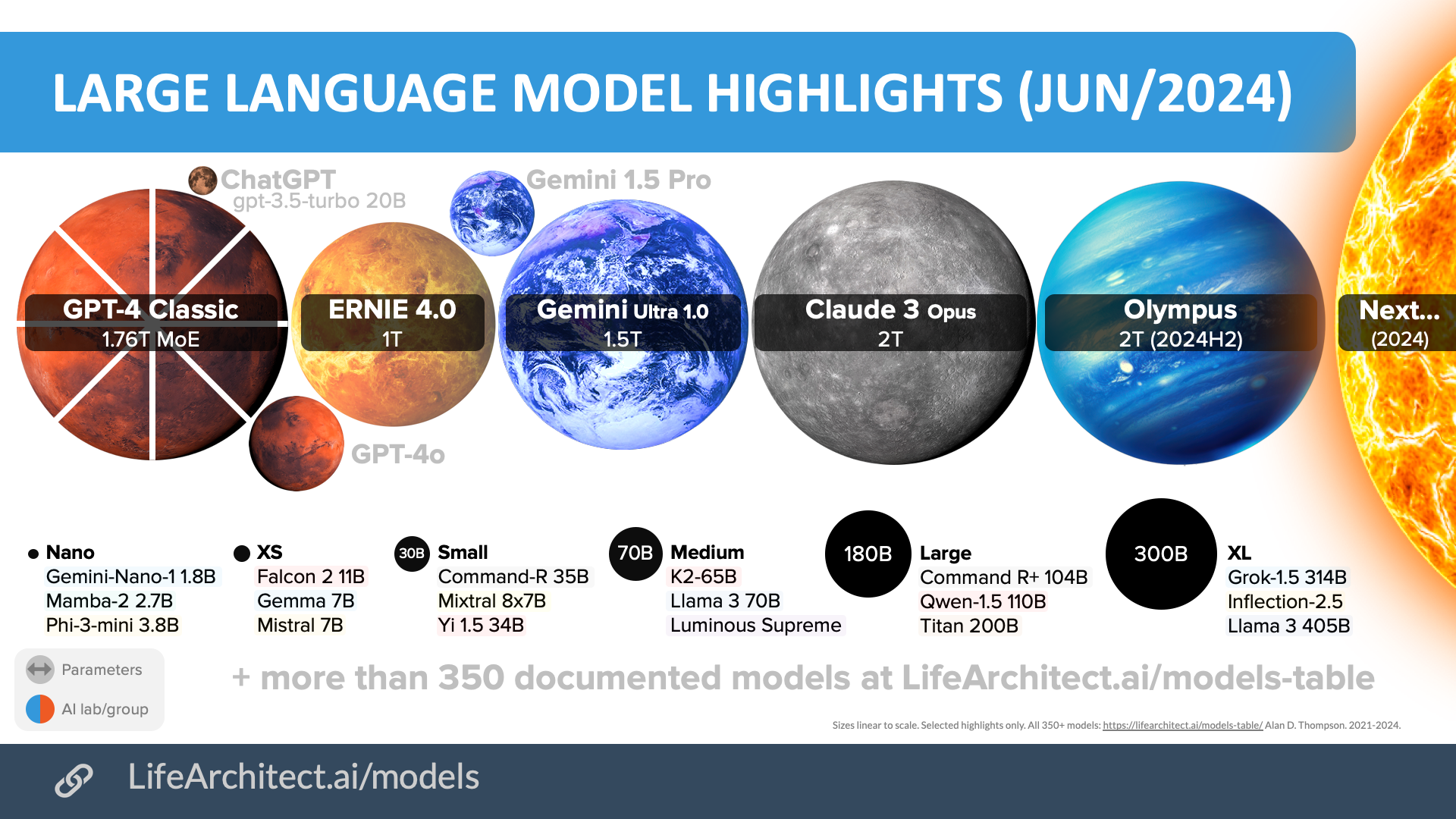
Permissions: Yes, you can use these visualizations anywhere, please leave the citation intact.
Older bubbles viz
Feb/2024
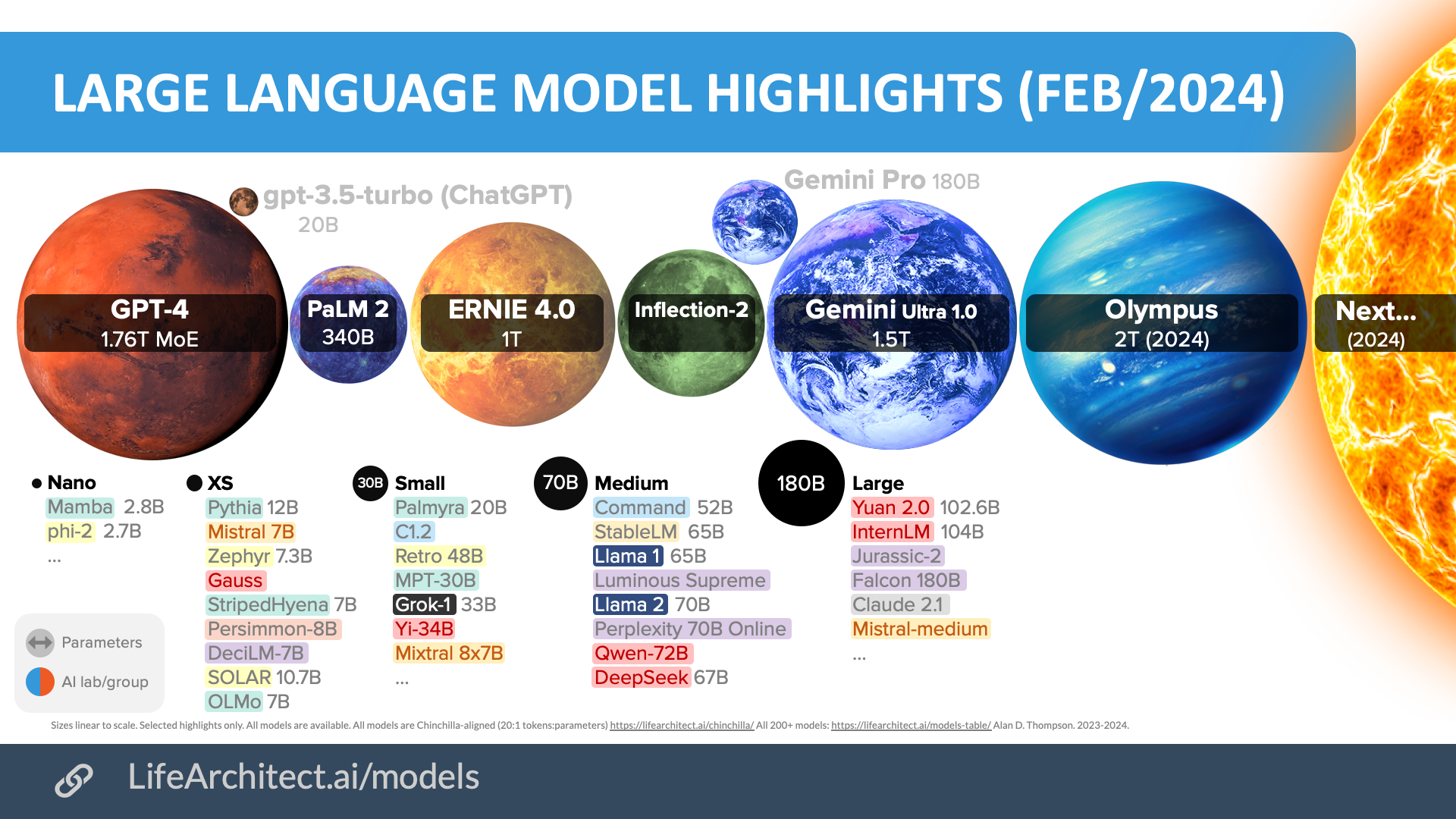
Nov/2023
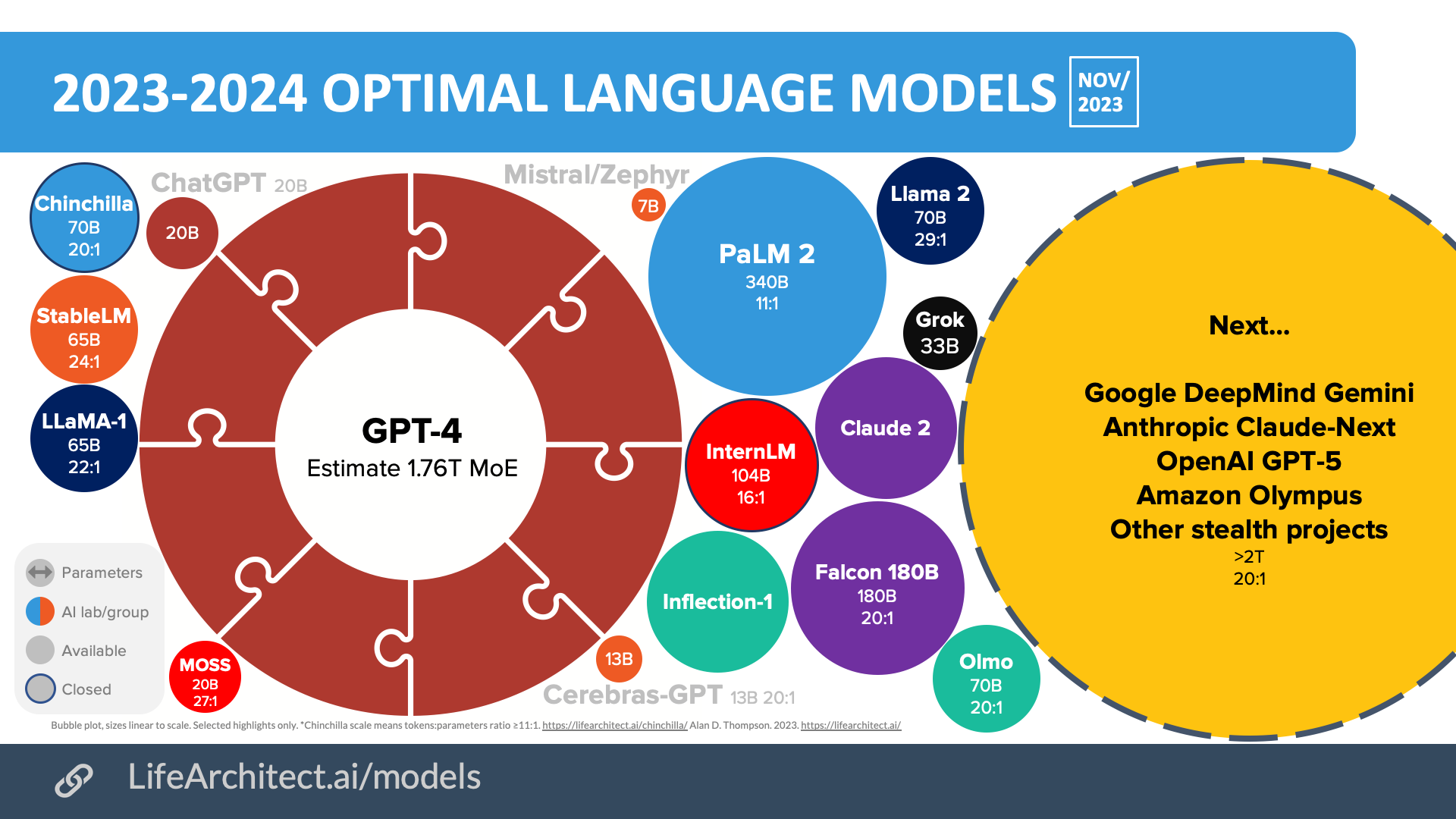
Permissions: Yes, you can use these visualizations anywhere, please leave the citation intact.
Mar/2023
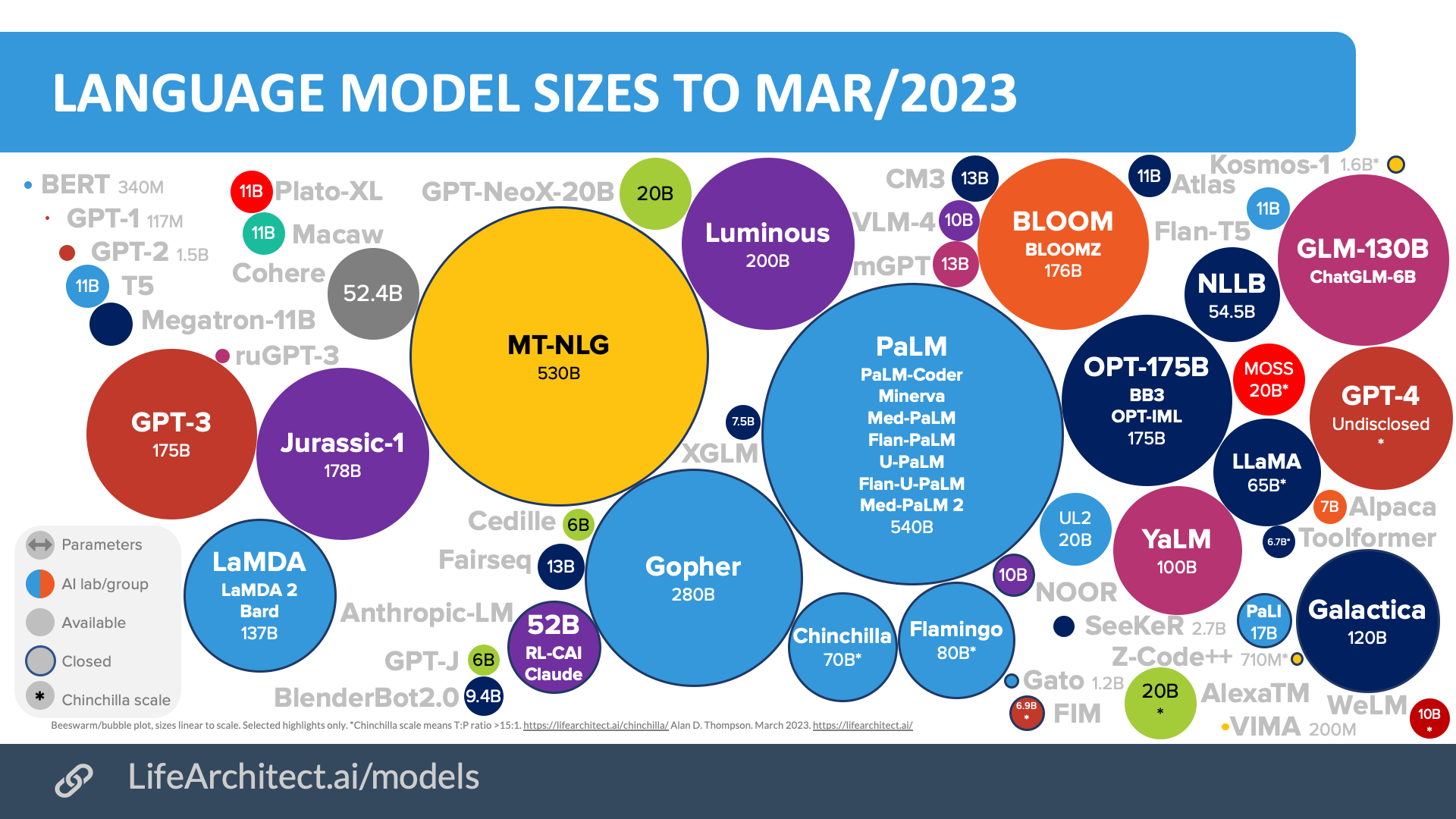
Apr/2022
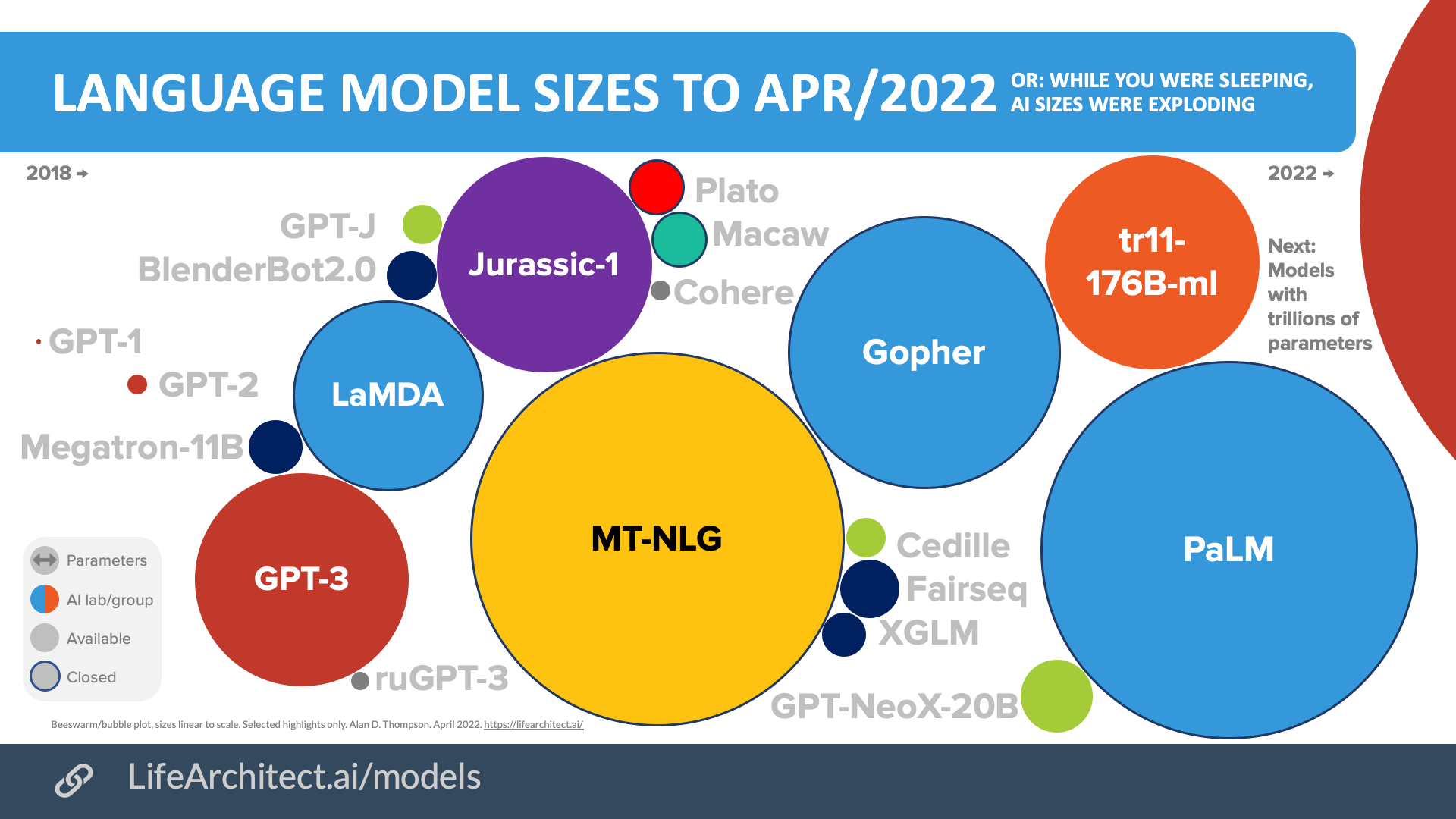
Summary of current models
Models Table
Summary of current models: View the full data (Google sheets)Billboard chart for language models
202406
ALScore: “ALScore” is a quick and dirty rating of the model’s power. The formula is: Sqr Root of (Parameters x Tokens) ÷ 300
. In mid-2023, any ALScore ≥ 1.0 is a powerful model.
Older Billboard charts for LLMs
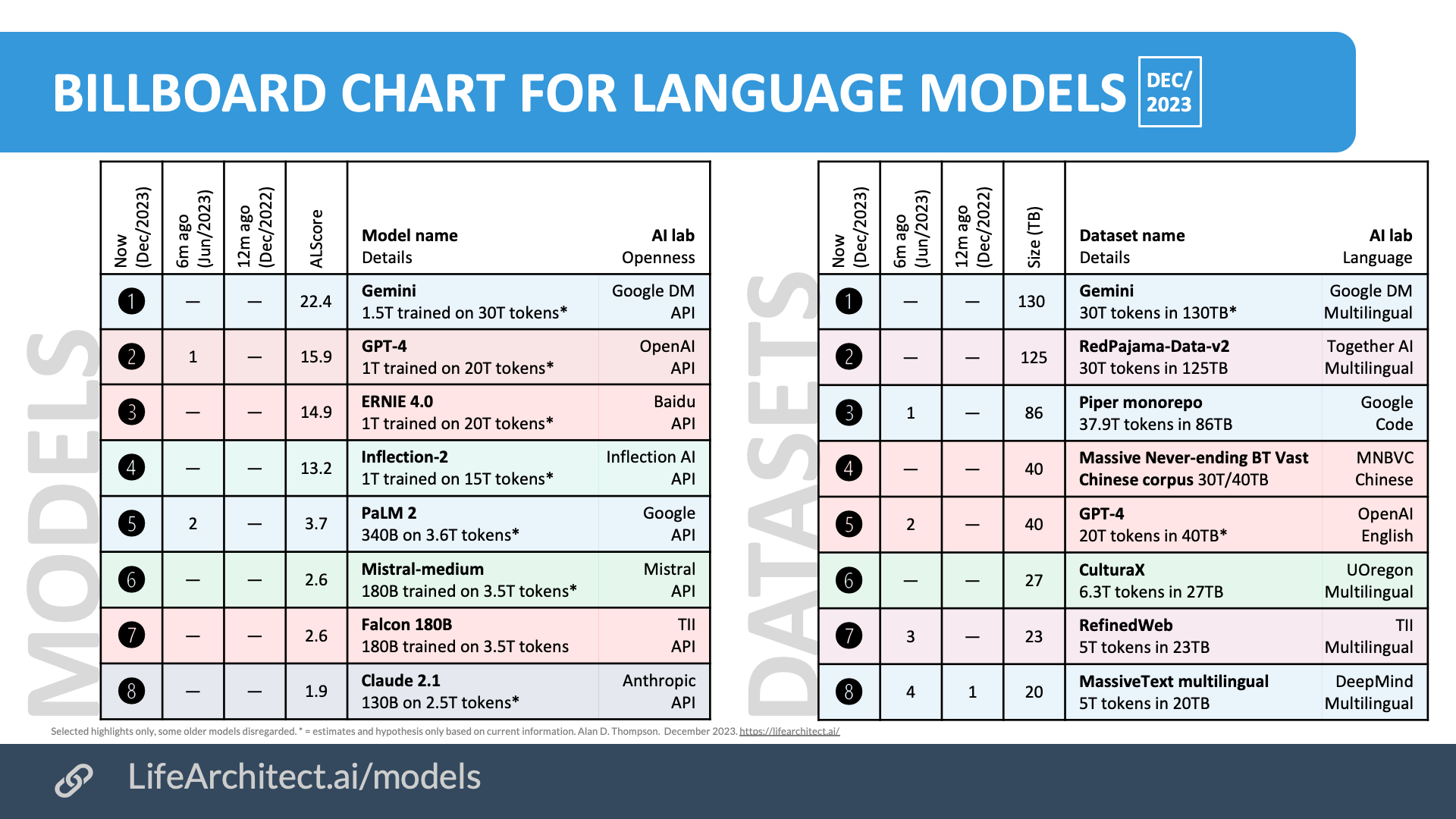
Sqr Root of (Parameters x Tokens) ÷ 300
. In mid-2023, any ALScore ≥ 1.0 is a powerful model.
202306
ALScore: “ALScore” is a quick and dirty rating of the model’s power. The formula is:
Sqr Root of (Parameters x Tokens) ÷ 300
. In mid-2023, any ALScore ≥ 1.0 is a powerful model.
Compute
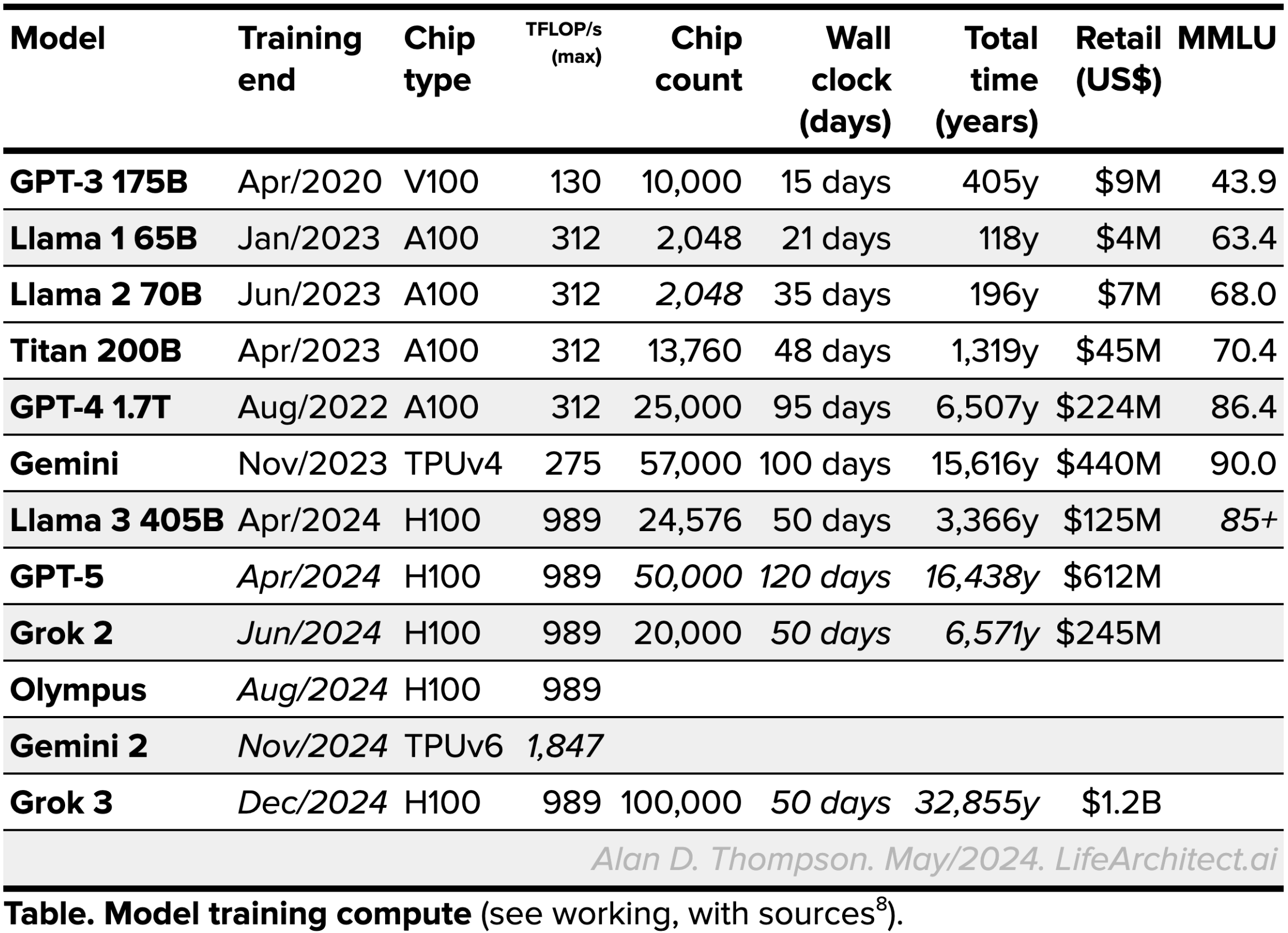
Context windows
Achievements unlocked: Emergent abilities of LLMs
Unpredictable abilities that have been observed in large language models but that were not present in simpler models (and that were not explicitly designed into the model) are usually called “emergent abilities”. Researchers note that such abilities “cannot be predicted simply by extrapolating the performance of smaller models”. (- wiki)
Download source (PDF)
Permissions: Yes, you can use these visualizations anywhere, please leave the citation intact.
Video
Large language models: API or on-premise
Using GPT-4 is like leasing a Boeing 787; you don’t own it, but it is the most powerful model. Hosting your own model like DeepSeek might be the next step down; a little Cessna. And then you can even build it yourself and keep it in your bedroom, that would be the paper plane, or the laptop models like Llama.
Increasing dataset sizes 2018-2023
Open the Datasets Table in a new tabWhat’s in my AI? A Comprehensive Analysis of Datasets Used to Train GPT-1, GPT-2, GPT-3, GPT-NeoX-20B, Megatron-11B, MT-NLG, and Gopher
Alan D. Thompson
LifeArchitect.ai
March 2022
26 pages incl title page, references, appendix.
GPT-3’s top 10 datasets by domain/source
Download source (PDF)
Contents: View the data (Google sheets)
Contents of GPT-3 & the Pile v1
Download source (PDF)
Contents: View the data (Google sheets)
Read detail of datasets within GPT-3 and the Pile v1, & see alternative viz
List of datasets in data models GPT-3, GPT-J, GPT-NeoX
GPT-3
What is in GPT-3? GPT-3 contains (sorted by most tokens/effective size):
- Common Crawl (www)
- WebText (Reddit links)
- Books2 (Libgen or similar)
- Books1/BookCorpus (Smashwords)
- Wikipedia (facts)
- -end of list-
The Pile v1
What is in the Pile v1? The Pile v1 contains (sorted by most tokens/effective size):
- Common Crawl (www)
- PubMed Central (papers)
- Books3 (Bibliotik tracker)
- WebText (Reddit links)
- ArXiv (papers)
- Github (code)
- FreeLaw (papers)
- Stack Exchange (discussion)
- USPTO Background (papers)
- PubMed Abstracts (papers)
- Gutenberg (books)
- OpenSubtitles (movies)
- Wikipedia (facts)
- DM Mathematics (papers)
- Ubuntu IRC (discussion)
- Books1/BookCorpus (Smashwords)
- EuroParl (formal discussion)
- HackerNews (discussion)
- YoutubeSubtitles (movies)
- PhilPapers (papers)
- NIH ExPorter (papers)
- Enron Emails (discussion).
- -end of list-
GPT-3 is sometimes misspelt as: GPT3, GPT 3, GPT three, GTP-3, GTP3, GTP 3, GTP three.
List of domains in the WebText dataset
- Google (www).
- Archive (www).
- Blogspot (blogs).
- GitHub (code).
- NYTimes (news).
- WordPress (blogs).
- Washington Post (news).
- BBC (news).
- The Guardian (news).
- eBay (goods).
- Pastebin (text).
- CNN (news).
- Yahoo! (news).
- Huffington Post (news).
Reference: https://github.com/openai/gpt-2/blob/master/domains.txt
List of domains in the C4 dataset
Common Crawl (C4)
What is in Common Crawl? Common Crawl includes (C4, cleaned/filtered, sorted by most tokens):
# | C4 (Filtered Common Crawl) contents with Wikipedia removed for dedup… | % of 156B | Tokens |
---|---|---|---|
1 | Google Patents (papers) | 0.48% | ~750M |
2 | The New York Times (news) | 0.06% | ~100M |
3 | Los Angeles Times (news) | 0.06% | ~90M |
4 | The Guardian (news) | 0.06% | ~90M |
5 | PLoS – Public Library of Science (papers) | 0.06% | ~90M |
6 | Forbes (news) | 0.05% | ~80M |
7 | HuffPost (news) | 0.05% | ~75M |
8 | Patents.com – dead link (papers) | 0.05% | ~71M |
9 | Scribd (books) | 0.04% | ~70M |
10 | The Washington Post (news) | 0.04% | ~65M |
11 | The Motley Fool (opinion) | 0.04% | ~61M |
12 | InterPlanetary File System (mix) | 0.04% | ~60M |
13 | Frontiers Media (papers) | 0.04% | ~60M |
14 | Business Insider (news) | 0.04% | ~60M |
15 | Chicago Tribune (news) | 0.04% | ~59M |
16 | Booking.com (discussion) | 0.04% | ~58M |
17 | The Atlantic (news) | 0.04% | ~57M |
18 | Springer Link (papers) | 0.04% | ~56M |
19 | Al Jazeera (news) | 0.04% | ~55M |
20 | Kickstarter (discussion) | 0.03% | ~54M |
21 | FindLaw Caselaw (papers) | 0.03% | ~53M |
22 | National Center for Biotech Info (papers) | 0.03% | ~53M |
23 | NPR (news) | 0.03% | ~52M |
… | and 1M+ more domains… | ~98.58% | ~153.8B |
A huge ‘thank you!’ to Drs Jesse Dodge and Maarten Sap from the Allen Institute for AI for the revised chart in the C4 paper.
You can also search for any domain in the C4 dataset using the index hosted by the Allen Institute for AI.
Contents of Chinese models
Download source (PDF)
Contents: View the data (Google sheets)
List of datasets in Chinese data models PanGu Alpha, Wudao 2.0
PanGu Alpha
What is in PanGu Alpha? PanGu Alpha contains (sorted by most tokens/effective size):
- Common Crawl (www)
- Public datasets: DuReader (discussion), Baidu QA (discussion), CAIL2018 (legal papers), SogouCA (news), and more…;
- News
- Encyclopedia: Baidu Baike (facts), Sogou Baike (facts), and more…;
- e-Books
- -end of list-
WuDao 2.0
WuDaoCorpora 1.0 (dataset) and Wudao 1.0 (model) were launched in March 2021.
WuDaoCorpora 2.0 (dataset) and Wudao 2.0 (model) were launched in June 2021 (at the 2021 BAAI conference).
WuDaoCorpora 2.0 is composed of three parts:
1. WDC-Text (3TB text), the world’s largest plain text dataset.
2. WDC-ImageCaption (90TB image and text), the world’s largest multimodal dataset.
3. WDC-Dialogue (180GB text), the world’s largest Chinese dialogue dataset.
WDC-Text (3TB text)
3TB of text data, with labelling. “20 strict cleaning rules used by WuDaoCorpora1.0, and derives high-quality datasets from more than 100TB of original web page data.”
WDC-ImageCaption (90TB image and text)
“Contains 630 million image and text pairs, with a total data volume of about 90TB, the largest in the world. Among them, 600 million is related to graphics and text, and 30 million is a specific description of the content of the image.”
WDC-Dialogue (180GB text)
“Contains 181GB of high-quality Chinese dialogue data, and the total number of dialogues reaches 1.4B… Cleaned up 180GB of high-quality dialogue data from 9TB of raw data.”
What is in Wudao 2.0? Wudao 2.0 contains:
WuDaoCorpora2 – Chinese text only:
- Zhihu (discussion)
- Baidu Baike (facts/encyclopedia)
- Sogou Baike (facts/encyclopedia)
- Baidu QA (discussion)
- Other*:
(*best guess only, sorted by most visits);
- Tencent QQ (messenger)
- Sohu (news)
- Sina Weibo (discussion)
- Sina Corporation (news)
- Xinhua News Agency (news)
- Chinese Software Dev Network (discussion)
- Global Times (news)
- Tianya Club (discussion)
- 17ok.com (finance discussion)
- BabyTree (parenting discussion)
- CNBlogs (software discussion)
- 6Rooms (news)
- NetEase (discussion)
- Hunan Rednet (news)
- Bilibili (video discussion)
- and more…
Sources:
https://www.aminer.cn/research_report/60b628f030e4d5752f50def3
https://baijiahao.baidu.com/s?id=1701360796163699362&wfr=spider&for=pc
“Corpora contains various data types including news, post bar comments (sic), encyclopedia information, etc. More specifically, WuDaoCorpora contains a 3 TB Chinese corpus collected from 822 million Web pages” (WuDaoCorpora paper, Tang et al, June 2021).
“For training of base model, we use a training set of 302GB, the distribution of these data is shown in Table 7” (Inverse Prompting paper, Tang et al, June 2021).
Wudao 2.0 is sometimes misspelt as: Wudao-2, Wudao 2, Wu dao 2.0, Woodao, Woo dao.
Chinese model names & dataset equivalent in English
PanGu Alpha: Launched by Huawei and others in April 2021.
Simplified Chinese: 盘古
Traditional Chinese: 盤古
Pinyin: Pán gǔ
Pronounced: pun-goo (rhymes with done tool)
English: Literal: ‘coil ancient’, first living being and the creator (coiled up in an egg).
Etymology: Mythical Chinese creation figure who emerged from a yin-yang egg and created the earth and sky (similar to the Christian creation story, and Pangu has been compared to Adam).
Wudao 2.0: Launched by the Beijing Academy of Artificial Intelligence (BAAI) and others in June 2021.
Simplified Chinese: 悟道
Traditional Chinese: 悟道
Pinyin: Wù dào
Pronounced: oo-dao (rhymes with tool now)
English: Literal: ‘Enlightenment’.
Etymology: Truth of the Dharma, the spiritual path.
Chinese dataset | ➔ | English dataset equivalent |
---|---|---|
Zhihu (discussion) | ➔ | Quora |
Baidu Baike (facts) (16M articles) | ➔ | English Wikipedia (7M articles) |
Sogou Baike (facts) | ➔ | English Wikipedia (7M articles) |
Baidu QA (discussion) | ➔ | Stack Exchange |
Tencent QQ (messenger) | ➔ | ICQ |
Sohu (news) | ➔ | NBC |
Sina Weibo (discussion) | ➔ | |
Sina Corporation (news) | ➔ | CNN |
Xinhua News Agency (news) | ➔ | CBS |
Chinese Software Dev Network (discussion) |
➔ | Stack Exchange |
Global Times (news) | ➔ | Washington Post |
Tianya Club (discussion) | ➔ | Yahoo! Groups |
17ok.com (finance discussion) | ➔ | Yahoo Finance |
BabyTree (parenting discussion) | ➔ | TheBump |
CNBlogs (software discussion) | ➔ | Hacker News |
6Rooms (news) | ➔ | Huffington Post |
NetEase (discussion) | ➔ | Blizzard |
Hunan Rednet (news) | ➔ | The New York Times |
Bilibili (video discussion) | ➔ | YouTube |
Language model sizes & predictions
Download source (PDF)
Sizes: View the data (Google sheets)
Facebook BlenderBot 2.0
Launched July 2021, BlenderBot 2.0 is pre-trained on (Reddit discussion), fine-tuned on ConvAI2, Empathetic Dialogues, and Wizard of Wikipedia (WoW) datasets. The two additional datasets are Multi-Session Chat and Wizard of the Internet (WizInt). To train for safety, it uses the BAD dataset. Finally—in realtime—it is able to add live results by ‘generating its own search queries, reading the results, and taking them into account when formulating a response.’
List of validation set domains in WizInt/BlenderBot 2.0
query
and query + "news"
) to respond to their partner in conversation. Search results were added to the conversation by the human 80.3% of the time. The resulting WizInt dataset (validation set of human conversations with search) is used as supervision for new queries in BlenderBot 2.0. That is, new conversations with BlenderBot 2.0 will generate new responses that may include live/realtime web search engine results.
Breakdown of most common domains used during search… (validation set breakdown). Shown is the most common 24.41%, there is a long tail of 1,233 other domains across the whole validation set.
Domain | % |
---|---|
Wikipedia | 8.56% |
IMDb | 3.08% |
Britannica | 2.28% |
Healthline | 0.84% |
All Recipes | 0.84% |
Rotten Tomatoes | 0.8% |
Ranker | 0.8% |
Genius | 0.76% |
Rolling Stone | 0.67% |
Live About | 0.63% |
The Spruce Eats | 0.55% |
The Guardian | 0.51% |
Biography | 0.51% |
Esquire | 0.42% |
The Spruce | 0.38% |
Men’s Health | 0.38% |
Book Series in Order | 0.38% |
Trip Savvy | 0.34% |
Forbes | 0.34% |
Thoughtco | 0.34% |
Wikihow | 0.34% |
WebMD | 0.34% |
Thrillist | 0.34% |
1,233 more domains… | 75.59% |
References for Blenderbot 2.0
Read the paper:
BlenderBot 2.0 (Facebook): Komeili et al (2021). Internet-Augmented Dialogue Generation. (PDF)
Facts on GPT-3
Think you’re a fast typer? In March 2021, GPT-3 was typing 3.1 million words per minute, non-stop, 24×7. With the general availability of the model, I expect that number is a lot higher now… (Nov/2021).
Per day = 4,500,000,000 (4.5 billion)
Per hour = 187,500,000 (187.5 million)
Per minute = 3,125,000 (3.125 million)
—
Every day, GPT-3 generates the equivalent of an entire US public library (80,000 books) of new content.
(“…more than 300 applications are now using GPT-3, and tens of thousands of developers around the globe are building on our platform. We currently generate an average of 4.5 billion words per day, and continue to scale production traffic.” (OpenAI blog, March 2021). Using an average of 55k words per book = 81,818 books per day. “In 2017, there were 9,045 public libraries in the United States with a total of 715 million books and serial volumes” (US stats) = 79,049 books per library.)
—
“GitHub says that for some programming languages, about 30% of newly written code is being suggested by the company’s AI programming tool Copilot.” (Axios, October 2021)
—
“The supercomputer developed for OpenAI [as of May 2020] is a single system with more than 285,000 CPU cores, 10,000 GPUs [assume NVIDIA Tesla V100 GPUs released May/2017, superseded by NVIDIA Ampère A100 GPUs in May/2020] and 400 gigabits per second of network connectivity for each GPU server.”
https://blogs.microsoft.com/ai/openai-azure-supercomputer/
—
“Training GPT-3 with 175 billion parameters would require approximately 288 years with a single V100 NVIDIA GPU.”
— https://arxiv.org/pdf/2104.04473.pdf
—
“…the model is a big black box, we can’t infer its beliefs.”
– InstructGPT paper, 2022.
—
“Despite the impending widespread deployment of foundation [language] models, we currently lack a clear understanding of how they work, when they fail, and what they are even capable of due to their emergent properties.”
– Stanford paper, 2021
—
Note that there are some challenges with writing books using GPT-3 due to the output token limits. 2,048 tokens is about…
- 1,430 words (token is 0.7 words).
- 82 sentences (sentence is 17.5 words).
- 9 paragraphs (paragraph is 150 words).
- 2.8 pages of text (page is 500 words).
There are clever ways to increase this output by feeding in the last/most important output to a new prompt.
Jurassic-1 (178B)
Launched 12/Aug/2021.
Our model was trained… on 300B tokens drawn from publicly available resources, attempting, in part, to replicate the structure of the training data as reported in Brown et al. (2020) [the GPT-3 dataset, which is detailed in the viz above at LifeArchitect.ai/models].
— AI21’s Jurassic-1 paper
BLOOM by BigScience & languages within LLMs
176B parameter multi-lingual model.
Trained in March-July 2022.
BLOOM = BigScience Language Open-source Open-access Multilingual.
The BigScience project for open research is a year-long initiative (2021-2022) targeting the study of large models and datasets. The goal of the project is to research language models in a public environment outside large technology companies. The project has 1,000 researchers from 60 countries and more than 250 institutions. The BigScience project was initiated by Thomas Wolf at Hugging Face.
https://github.com/bigscience-workshop/bigscience/tree/master/train/tr11-176B-ml
Languages: View the data (Google sheets)
M6 by Alibaba
Multi-Modality to MultiModality Multitask Mega-transformer (M6)
From 100B to 1T to 10T parameters in less than a year!
“M6-Corpus for pretraining in Chinese, which consists of over 1.9TB image and 292GB text. The dataset has large coverage over domains, including encyclopedia, question answering, forum discussion, common crawl, etc”
https://arxiv.org/pdf/2103.00823.pdf
Megatron
Due to the complexity of this transformer and related language models, Megatron has its own page showing a summary of timeline, labs involved, and other details.
InstructGPT by OpenAI one-pager
* The initialism ‘HHH’ was coined by Anthropic, and demonstrated in InstructGPT.
WebGPT by OpenAI sample question set
Contents: View the data (Google sheets)
PaLM by Google: Explaining jokes + Inference chaining
A full report on PaLM (and Pathways) was released Aug/2022.
Luminous by Aleph Alpha
The Luminous text model was announced at a conference in Nov/2021, where the parameter count for Luminous (assuming Luminous-World, in progress as of Apr/2022) was said to be 200B.
The announced luminous model uses up to 200 billion parameters and is considered to be just as powerful in the text part as GPT, whose third version includes up to 175 billion parameters. In contrast to the American counterpart, luminous can be combined with any number of images, the model is available in five languages (German, English, French, Italian, Spanish) and has been trained in the European cultural context.
— Heise, translated.
# | Model name | Token count* |
---|---|---|
1 | Luminous Base | 13B |
2 | Luminous Extended | 30B |
3 | Luminous Supreme | 70B |
4 | Luminous Supreme Control | 70B |
5 | Luminous World | 200B (from presentation) |
* sizes confirmed via https://crfm-models.stanford.edu/static/help.html
DeepMind’s models
DeepMind’s models are: Gopher, Chinchilla, Flamingo, Gato (cat), Sparrow, Dramatron, and SFT-Utilitarian. Chinchilla has been fine-tuned and prompted for Sparrow and SFT-Utilitarian, and prompted for Dramatron.
Sep/2022: Sparrow (based on Chinchilla 70B)
Google Imagen
Google Imagen has 2B image parameters + 1B upscale parameters + 4.6B LLM parameters (text encoding) via T5-XXL. Google Imagen was released by the Google Research and Google Brain teams in Toronto, Canada.
BriVL by RUC, China (Jun/2022)
BriVL seems to be mainly a publicity stunt, to drive marketing to Beijing’s pursuit of being an AI leader.
BriVL a year ago (Mar/2021)
>”[In Mar/2021] The first version of our BriVL model has 1 billion parameters, which is pretrained on the RUC-CAS-WenLan dataset with 30 million image-text pairs…In the near future, our BriVL model will be enlarged to 10 billion parameters, which will be pre-trained with 500 million imagetext pairs.” — https://arxiv.org/pdf/2103.06561.pdf
BriVL today (Jun/2022)
“[In Jun/2022] With 112 NVIDIA A100 GPUs in total, it takes about 10 days to pre-train our BriVL model over our WSCD of 650 million image-text pairs.” – Nature Communications
Conclusion
CLIP was 400M image-text pairs trained to 63M parameters. DALL-E had 250M pairs and 12B Parameters. So… BriVL is a nice evolution here.
Marketing quotes
The most interesting parts of the paper were the bombastic and flowery quotes around artificial general intelligence (AGI).
First, compare this quote from the cautious and mindful open-source AI lab, EleutherAI, in their paper on GPT-NeoX-20B:
We believe that Transformative Artificial Intelligence (TAI) is approaching… recent increases in the capabilities of large language models (LLMs) raises the possibility that the first generation of transformatively powerful AI systems may be based on similar principles and architectures as current large language models like GPT. This has motivated a number of research groups to work on “prosaic alignment”, a field of study that considers the AI alignment problem in the case of TAI being built primarily with techniques already used in modern ML. We believe that due to the speed of AI progress, there is a significant chance that this assumption is true, and, therefore, that contributing and enabling contributions to prosaic alignment research will have a large impact. – EleutherAI, 20B paper, Feb/2022
Next, compare the carefulness above with the Chinese BriVL paper:
– “…we demonstrate that strong imagination ability is now possessed by our foundation model. We believe that our work makes a transformative stride towards AGI, from our common practice of “weak or narrow AI” to that of “strong or generalized AI”.”
– “BriVL possesses strong capability of imagination given a complicated sentence as prompt.”
– “…even hints of common sense reasoning ability of our BriVL.”
– “…by effectively fusing the complex human emotions and thoughts from those weakly correlated image-text pairs, our BriVL is made more cognitive and general (i.e., much closer to AGI).”
Perceiver AR by DeepMind (Jun/2022)
Perceiver AR (autoregressive), modality-agnostic architecture… can directly attend to over a hundred thousand tokens, enabling practical long-context density estimation.
AlexaTM 20B by Amazon Alexa AI (Aug/2022)
See LifeArchitect.ai report card.
Dataset: multilingual Wiki + mC4 only.
Training cost at standard rate:
====
16x AWS p4d.24xlarge compute instances
(8x GPUs each = 128x NVIDIA A100 GPUs)
= $32.77/hr on-demand each
= $524.32/hr on-demand total
x
2880 hours (120 days)
= $1,510,041.60
====
DeepL
most publicly available translation systems are direct modifications of the Transformer architecture. Of course, the neural networks of DeepL also contain parts of this architecture, such as attention mechanisms. However, there are also significant differences in the topology of the networks that lead to an overall significant improvement in translation quality over the public research state of the art. We see these differences in network architecture quality clearly when we internally train and compare our architectures and the best known Transformer architectures on the same data.
Most of our direct competitors are major tech companies, which have a history of many years developing web crawlers. They therefore have a distinct advantage in the amount of training data available. We, on the other hand, place great emphasis on the targeted acquisition of special training data that helps our network to achieve higher translation quality. For this purpose, we have developed, among other things, special crawlers that automatically find translations on the internet and assess their quality. In public research, training networks are usually trained using the “supervised learning” method. The network is shown different examples over and over again. The network repeatedly compares its own translations with the translations from the training data. If there are discrepancies, the weights of the network are adjusted accordingly. We also use other techniques from other areas of machine learning when training the neural networks. This also allows us to achieve significant improvements… we (like our largest competitors) train translation networks with many billions of parameters. – https://www.deepl.com/en/blog/how-does-deepl-work (Oct/2021)
Code Generation models (Sep/2022)
Data/compute-optimal (Chinchilla) heatmap (Nov/2022)
There is a new Chinchilla scaling page.
GPT-3.5 + ChatGPT
GPT-3.5 + ChatGPT: An illustrated overview.
2022 model count
Baidu ERNIE 3.0 Titan 260B (Wenxin)
Due to the complexity of this transformer and related language models, ERNIE has its own page.
Together AI’s RedPajama dataset
Count | Dataset | Tokens (B) | % | Raw size (GB) |
---|---|---|---|---|
1 | Common Crawl | 878 | 73.2% | 2,927 |
2 | C4 | 175 | 14.6% | 583 |
3 | GitHub | 59 | 4.9% | 197 |
4 | Books | 26 | 2.2% | 87 |
5 | ArXiv | 28 | 2.3% | 93 |
6 | Wikipedia | 24 | 2.0% | 80 |
7 | StackExchange | 20 | 1.7% | 67 |
Totals | 1210 | 4,033GB |
Rounded. Derived in italics.
Source: https://github.com/togethercomputer/RedPajama-Data
Compare with other major datasets in my paper: What’s in my AI?
Compare with Google’s Infiniset dataset (LaMDA/Bard).
Compare with OpenAI’s GPT-4 dataset.
Imitation models & synthetic data; from Alpaca to Phoenix
Imitation models includes LLaMA-based models like Alpaca, Dolly 2.0, BELLE, Vicuna, Koala, and Phoenix.
The viz below (big version) is from the paper ‘A Survey of Large Language Models‘ by Zhao et al, p10, first released 31/Mar/2023, updated to 29/Jun/2023 and with a smaller version via their GitHub.
The table below is from the paper ‘Phoenix: Democratizing ChatGPT across Languages‘ by Chen et al, pp4, released 20/Apr/2023.
And the figure below compares the ‘relative response quality’ of selected laptop models and dialogue models (like Bard and ChatGPT) as assessed by GPT-4 (pp11).
I am very much against smaller models trained on synthetic data produced by larger models. Here are two important sources:
imitation models close little to none of the gap from the base LM to ChatGPT on tasks that are not heavily supported in the imitation data… these performance discrepancies may slip past human raters because imitation models are adept at mimicking ChatGPT’s style but not its factuality… model imitation is a false promise: there exists a substantial capabilities gap between open and closed LMs… the highest leverage action for improving open-source models is to tackle the difficult challenge of developing better base LMs, rather than taking the shortcut of imitating proprietary systems.. (UC Berkeley, May/2023)
and
use of model-generated content in training causes irreversible defects in the resulting models, where tails of the original content distribution disappear. We refer to this effect as model collapse (Oxford, Cambridge, May/2023)
Tiny models + Apple UniLM 34M
See original posts about this model:
https://jackcook.com/2023/09/08/predictive-text.html
https://github.com/jackcook/predictive-spy
Get The Memo
by Dr Alan D. Thompson · Be inside the lightning-fast AI revolution.Bestseller. 10,000+ readers from 142 countries. Microsoft, Tesla, Google...
Artificial intelligence that matters, as it happens, in plain English.
Get The Memo.

This page last updated: 21/Jul/2024. https://lifearchitect.ai/models/↑