Get The Memo.
Summary
Organization | OpenAI |
Model name | GPT-6 |
Internal/project name | |
Model type | Multimodal |
Parameter count | Trillions of parameters |
Dataset size (tokens) | Quadrillions of tokens |
Training data end date | Oct/2024 (est) + Real-time learning |
Training start date | Dec/2024 (est) |
Training end/convergence date | Jun/2025 (est) |
Training time (total) | 100,000+ H100s and GB200s…![]() |
Release date (public) | 2025 |
Paper | – |
Playground | – |

2025 frontier AI models + highlights
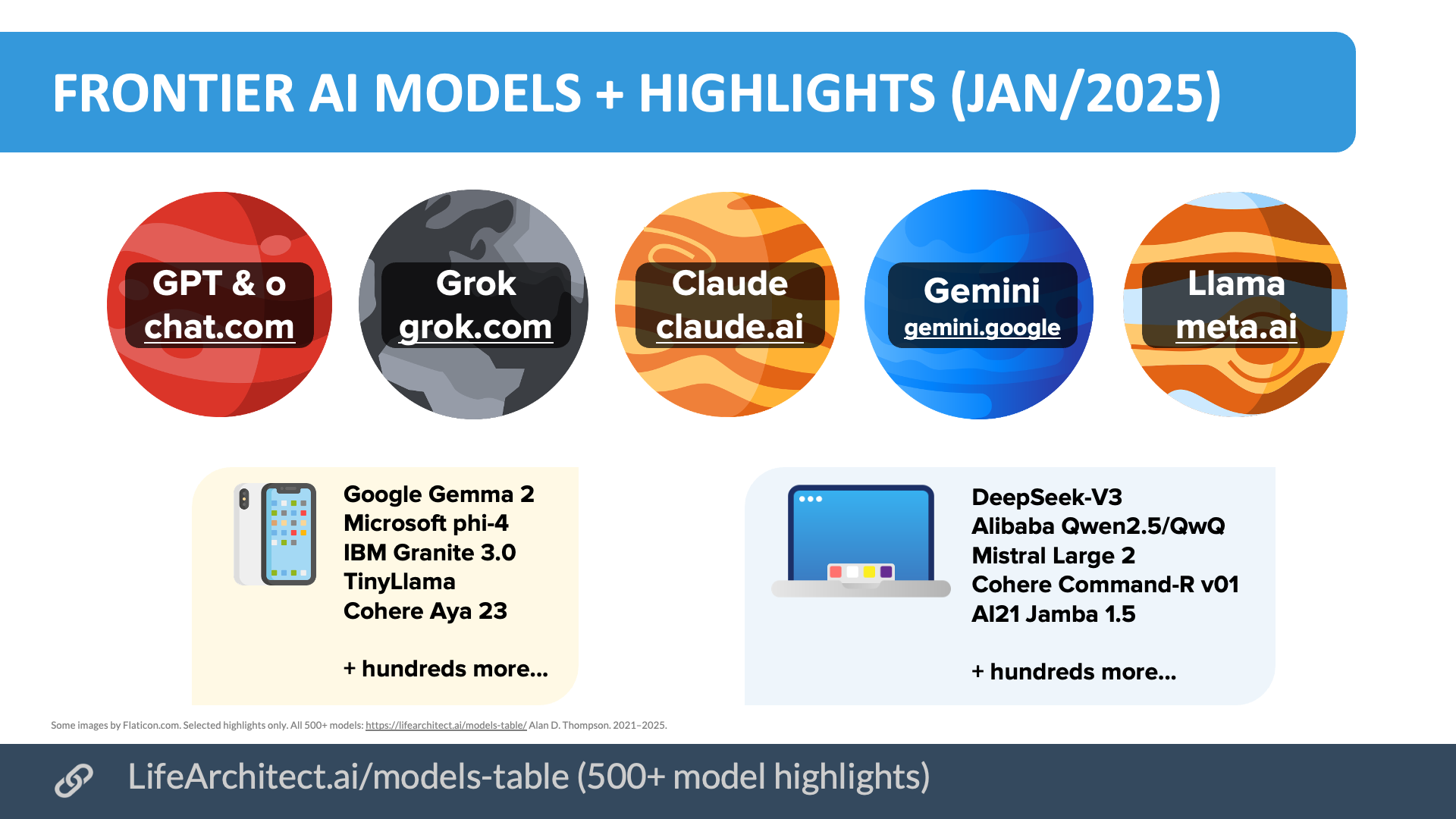
Permissions: Yes, you can use these visualizations anywhere, please leave the citation intact.
Older bubbles viz

Permissions: Yes, you can use these visualizations anywhere, please leave the citation intact.
Oct/2024
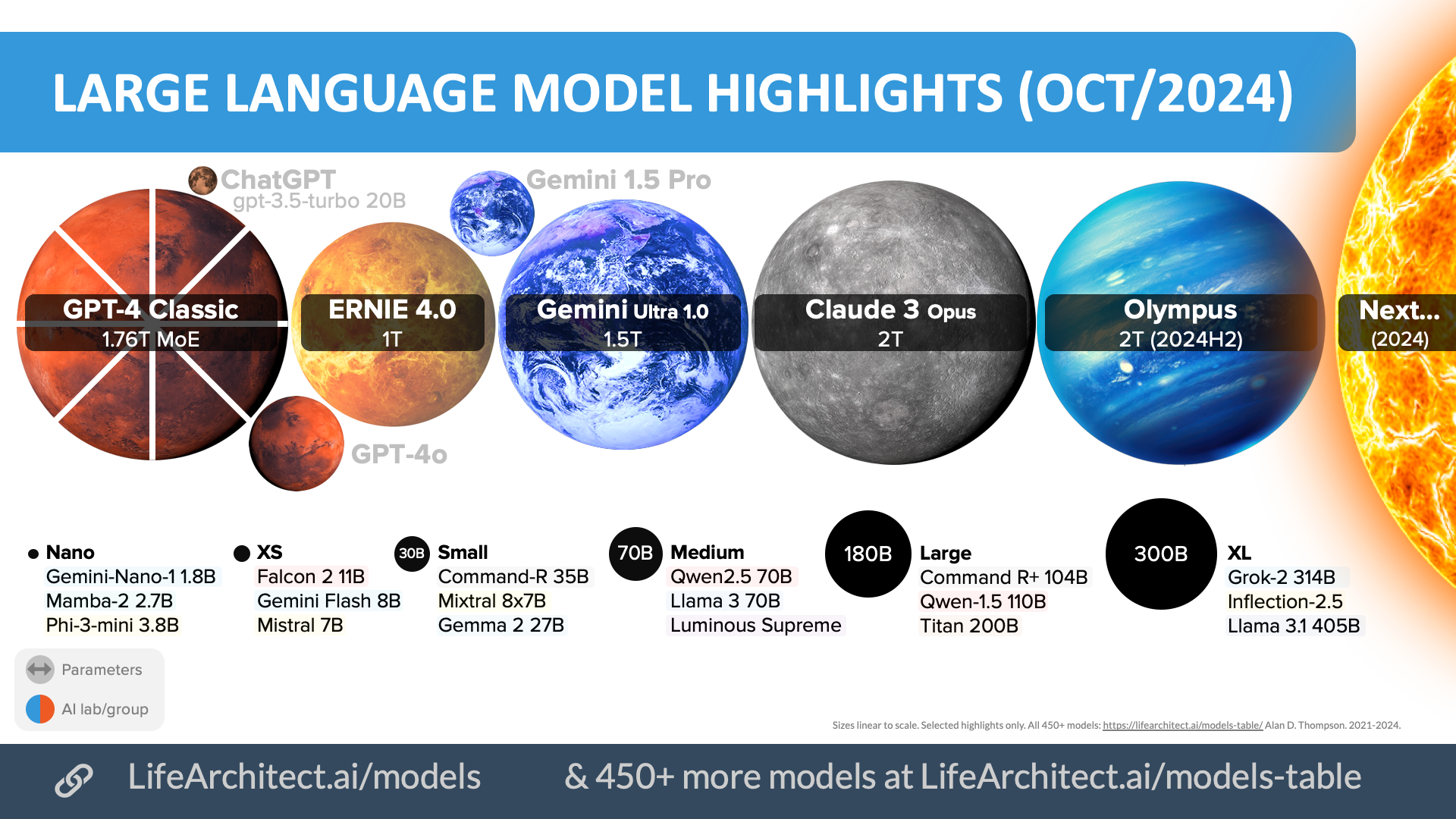
Permissions: Yes, you can use these visualizations anywhere, please leave the citation intact.
Feb/2024
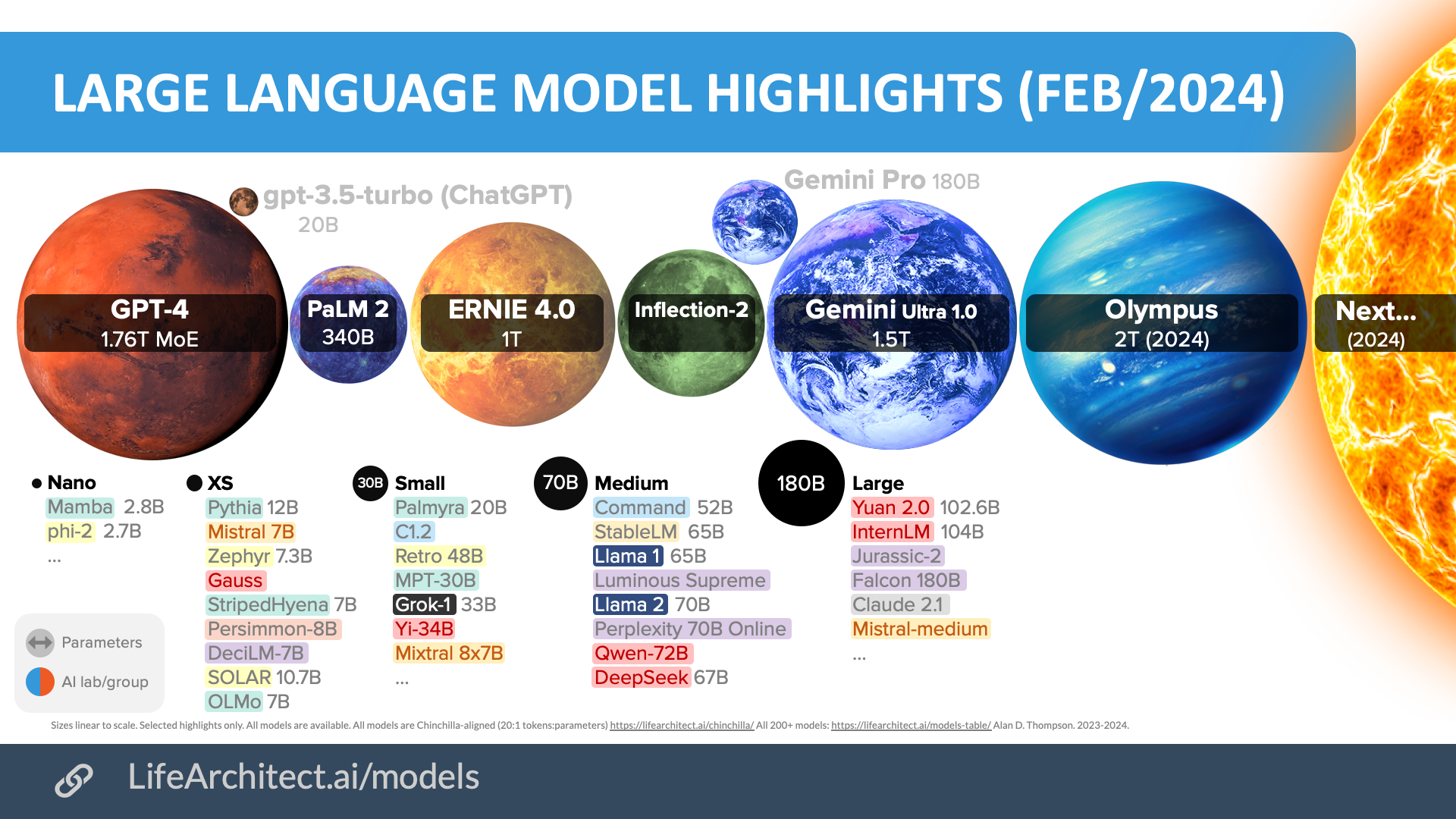
Nov/2023
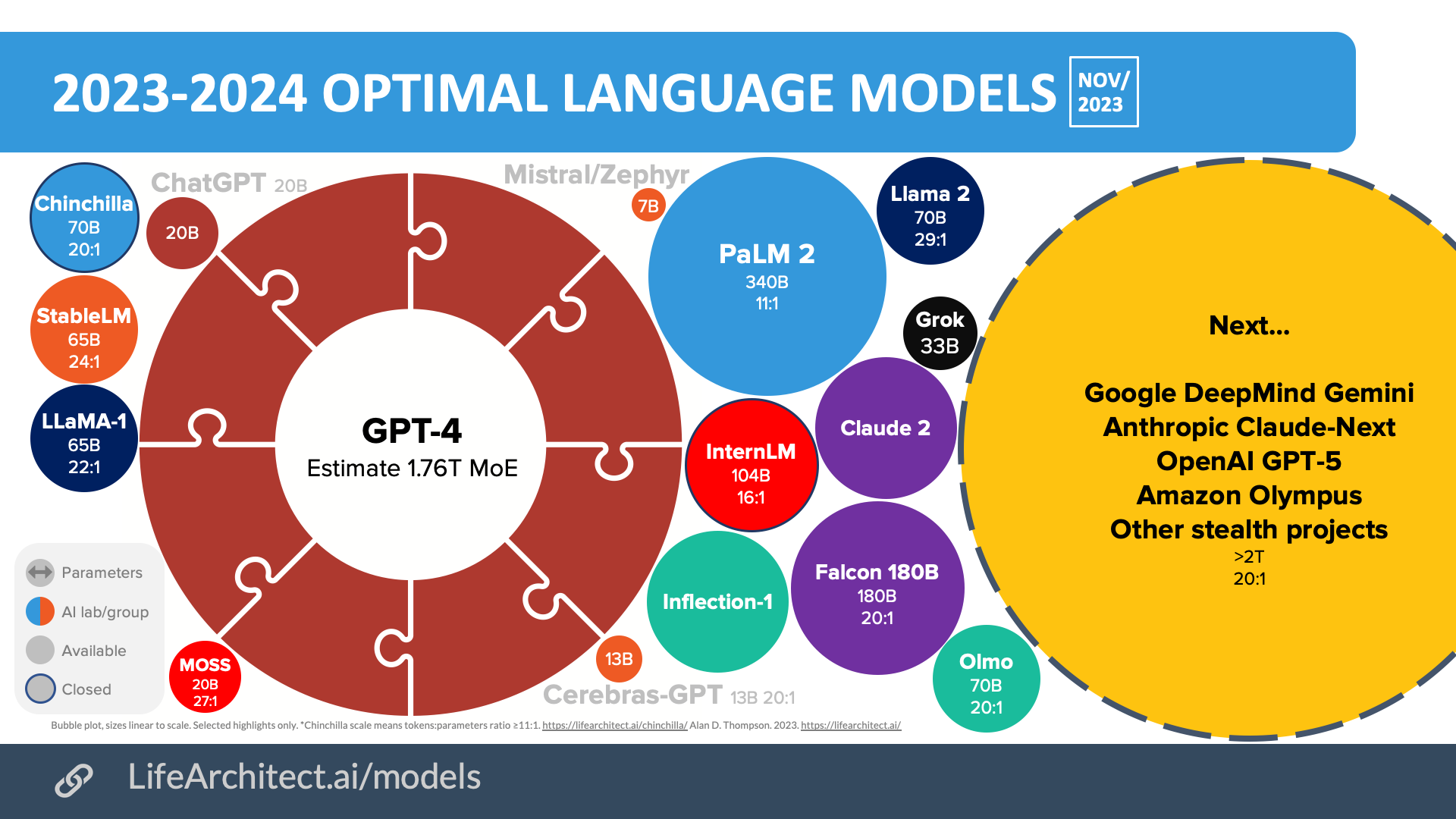
Permissions: Yes, you can use these visualizations anywhere, please leave the citation intact.
Mar/2023
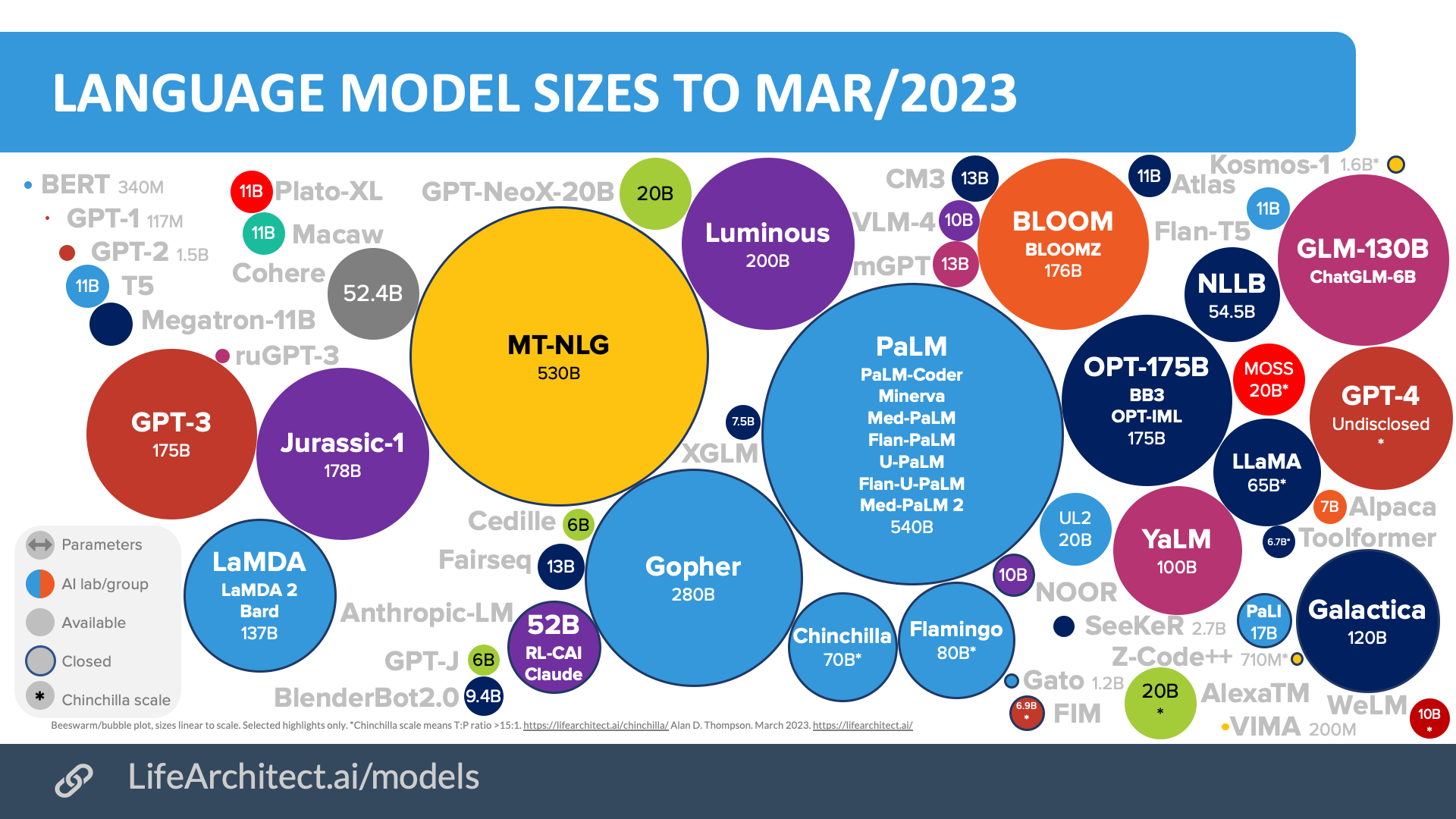
Apr/2022
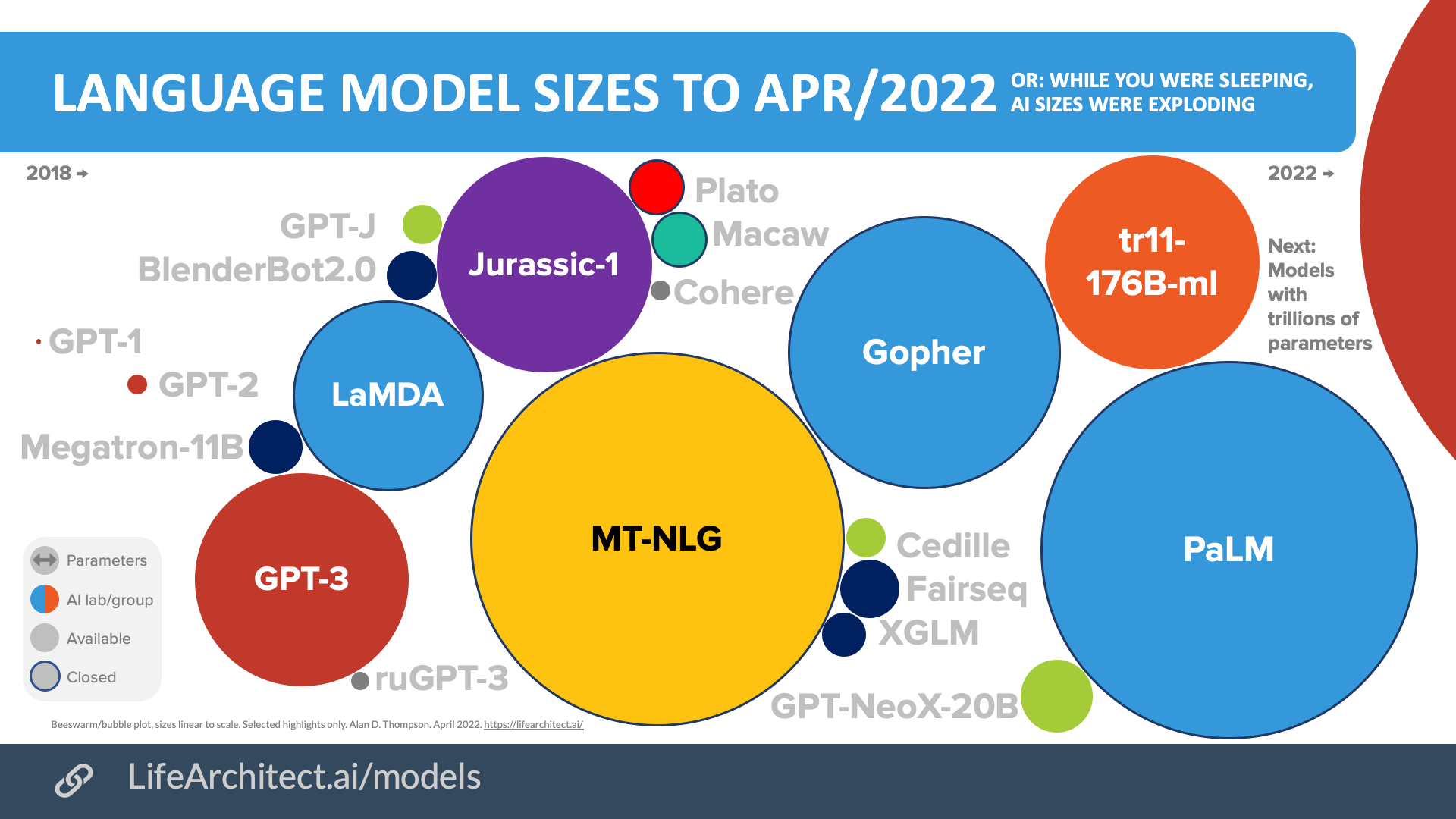
GPT-6 Updates
17/Oct/2024: OpenAI CEO: ‘We had thought at one point about, “It doesn’t fit perfectly but maybe we’ll call this [o1 model] GPT-5.” But this is a new paradigm. It’s a different way to use a model, it’s good at different things. It takes a long time for hard problems, which is annoying, but we’ll make that better. But it can do things that the GPT series just didn’t.’ (12/Sep/2024 @ UMich)
8/Oct/2024: First DGX B200s delivered to OpenAI for GPT-6 (2025) ‘Look what showed up at our doorstep. Thank you to NVIDIA for delivering one of the first engineering builds of the DGX B200 to our office.’ (Twitter)
4/Oct/2024: OpenAI CFO to CNBC:
10:45
There is no denying that we’re on a scaling law right now where orders of magnitude matter. The next model [GPT-5] is going to be an order of magnitude bigger, and the next one, and on and on.12:10
Interviewer: What about GPT-5? When can we expect that?Sarah: …We’re so used to technology that’s very synchronous, right? You ask a question, boom, you get an answer straight back. But that’s not how you and I might talk, right? If you called me yesterday, you might say, “Hey, prep for this.” I might take a whole day. And think about models that start to move that way, where maybe it’s much more of a long-horizon task—is the phrase we use internally. So it’s going to solve much harder problems for you, like even on the scale of things like drug discovery. So sometimes you’ll use it for easy stuff like “What can I cook for dinner tonight that would take 30 minutes?” And sometimes it’s literally “How could I cure this particular type of cancer that is super unique and only happens in children?” There’s such a breadth of what we can do here. So I would focus on these types of models and what’s coming next. It’s incredible.
Sidenote: An order of magnitude bigger than 1.76 trillion parameters MoE is 17.6 trillion parameters MoE, or around 3.5T parameters dense.
4/Sep/2024: Samsung President/Head of Memory Business Dr Jung-Bae Lee showed this slide about GPT-5 at SemiCon Taiwan: GPT-5 as 3–5T parameters, trained on 7,000× NVIDIA B100.
27/Aug/2024: TTT-Learning by Stanford, UC, and Meta: “Learning During Use: Usually, AI models are trained once and then used without changing. TTT [Test-Time Training] models continue to learn and adapt while they’re being used, helping them understand each specific text better. The researchers explain, “Even at test time, our new layer still trains a different sequence of weights W₁, …, Wₜ for every input sequence” [Section 2.1]. This dynamic adaptation is a key feature of TTT.” (Analysis, Paper)
16/Aug/2024: Mikhail Parakhin Microsoft CEO, Advertising and Web Services: ‘In order to get some meaningful improvement, the new model should be at least 20x bigger. Training takes at least 6 months, so you need a new, 20x bigger datacenter, which takes about a year to build (actually much longer, but there is pipelining).’
Note: This is in line with my estimates for GPT-5 as covered in my paper: What’s in GPT-5?
In order to get some meaningful improvement, the new model should be at least 20x bigger. Training takes at least 6 months, so you need a new, 20x bigger datacenter, which takes about a year to build (actually much longer, but there is pipelining).
— Mikhail Parakhin (@MParakhin) August 16, 2024
19/Jun/2024: Microsoft AI chief Mustafa Suleyman: “I think that it’s going to require not just one but two orders of magnitude more computation to train the models. So, we’re not looking at GPT-5 but more like GPT-6 scale models. I believe we’re talking about two years before we have systems that can truly take action.” Interview 19/Jun/2024.
28/May/2024: “OpenAI has recently begun training its next frontier model and we anticipate the resulting systems to bring us to the next level of capabilities on our path to AGI. While we are proud to build and release models that are industry-leading on both capabilities and safety, we welcome a robust debate at this important moment.” (OpenAI blog)
23/May/2024: Microsoft compares frontier models to marine wildlife: shark (GPT-3), orca (GPT-4), whale (GPT-5), blah blah blah. Video (link):
I definitely don’t recommend overanalyzing what Microsoft has said, but if we did want to overanalyze(!), it might look something like this. Note that I used Claude 3 Opus for working, and the delta always uses shark (or GPT-3) as the baseline:
Characteristic | Shark | Orca | Whale |
---|---|---|---|
Body weight (kg) | 500 | 5,000 | 150,000 |
Delta | 1x | 10x | 300x |
Brain Weight (kg) | 0.05 | 5.6 | 7.8 |
Delta | 1x | 112x | 156x |
Neurons | 100 million | 11 billion | 200 billion |
Delta | 1x | 110x | 2,000x |
Synapses | 10^13 (10 trillion) | 10^15 (1 quadrillion) | 10^16 (10 quadrillion) |
Delta | 1x | 100x | 1,000x |
Let’s use body weight, as it’s the closest match to the known deltas (GPT-3 ➜ GPT-4) to predict GPT-5:
Characteristic | GPT-3 | GPT-4 | GPT-5 |
---|---|---|---|
Parameters | 175B | 1,760B (1.76T) | 52,500B (52.5T) |
Delta | 1x | 10x | 300x |
Pretty close. Jensen/NVIDIA reckons we easily have hardware to train a 27T parameter model using just 20,000 GB200 chips, and we know Microsoft has added another 150,000 of the ‘older’ H100s, so 50T parameters certainly isn’t out of the question…
26/Mar/2024: Former Google engineer Kyle Corbitt (Twitter, 26/Mar/2024): ‘Spoke to a Microsoft engineer on the GPT-6 training cluster project. He kvetched about the pain they’re having provisioning infiniband-class links between GPUs in different regions. Me: “why not just colocate the cluster in one region?” Him: “Oh yeah we tried that first. We can’t put more than 100K H100s in a single state without bringing down the power grid.” 🤯’
Spoke to a Microsoft engineer on the GPT-6 training cluster project. He kvetched about the pain they're having provisioning infiniband-class links between GPUs in different regions.
Me: "why not just colocate the cluster in one region?"
Him: "Oh yeah we tried that first. We…— Kyle Corbitt (@corbtt) March 25, 2024
NVIDIA H100 @ 700W x 100,000 = 70,000,000W = 70,000kW = 70MW. Claude 3 Opus said:
70 megawatts (MW) is a significant amount of power. To put it in perspective, this is roughly the amount of electricity needed to power a small city or a large town.
For example, a city with around 70,000 households, assuming an average household consumes about 1 kW consistently, would require approximately 70 MW of power.
And, as detailed in The Memo edition 2/Dec/2023, Microsoft does indeed have an extra 150,000 H100s as of 2023:
18/Mar/2024: CNBC: NVIDIA said Amazon Web Services would build a server cluster with 20,000 GB200 chips. NVIDIA said that the system can deploy a 27-trillion-parameter model… (18/Mar/2024).
17/Mar/2024: Rumors that GPT-5 will be released mid-2024 (rather than the previous timeline of being delayed until after the Nov/2024 US elections).
14/Mar/2024: OpenAI CEO on GPT-5 to Korean media (14/Mar/2024):
“I don’t know when GPT-5 will be released, but it will make great progress as a model that takes a leap forward in advanced inference functions. What are the limitations of GPT? There are many questions as to whether it exists, but I will confidently say ‘no.’ We are confident that there are no limits to the GPT model and that if sufficient computational resources are invested, it will not be difficult to build AGI that surpasses humans.
“Many startups assume that the development of GPT-5 will be slow because they are happier with only a small development (since there are many business opportunities) rather than a major development, but I think it is a big mistake. When this happens, as often happens, it will be ‘steamrolled’ by the next generation model. In the past, we had a very broad picture of everything happening in the world and were able to see things that we couldn’t see from a narrow perspective, unfortunately, these days, we are completely focused on AI (AI all of the time at full tilt), so there is a different perspective. It is difficult to have.
“Other than thinking about the next generation AI model, the area where I spend the most time recently is ‘building compute,’ and I am increasingly convinced that computing will become the most important currency in the future. [But the world,] they have not planned enough computing and are not facing this problem, so there is a lot of concern about what is needed to build a huge amount of computing as cheaply as possible.
“What I am most excited about from AGI is that the faster we develop AI through scientific discoveries, the faster we will be able to find solutions to power problems by making nuclear fusion power generation a reality. Scientific research through AGI will lead to sustainable economic growth. I think it is almost the only driving force and determining factor.
“In the long run, there will be a shortage of human-generated data. For this reason, we need models that can learn more with less data.
8/Mar/2024: The Memo GPT-5 convergence date due mid-March 2024 (8/Mar/2024) GPT-5 which should have started training before Dec/2023 (OpenAI CEO under oath 16/May/2023: ‘We are not currently training what will be GPT-5; we don’t have plans to do it in the next six months [to 16/Nov/2023]’), and so 120 days later would be due to complete that training next Friday 15 March 2024. For safety, I expect the GPT-5 public release date to be after the November 2024 US elections.
31/Jan/2024: The Memo: Exclusive: GPT-5 and gold datasets (31/Jan/2024)
When raising a child prodigy, should we provide more learning and experiences or higher-quality learning and experiences?
When training frontier models like GPT-5, should we use more data or higher-quality data?
In Jun/2021, I published a paper called ‘Integrated AI: Dataset quality vs quantity via bonum (GPT-4 and beyond)’. It explored high-quality data aligned with ‘the ultimate good’ (in Latin, this is ‘summum bonum’).
OpenAI’s CEO recently spoke at a number of big venues including the 54th annual meeting of the World Economic Forum (WEF) at Davos-Klosters, Switzerland from 15th to 19th January 2024. He was recorded as making a very interesting comment:
As models become smarter and better at reasoning, we need less training data. For example, no one needs to read 2000 biology textbooks; you only need a small portion of extremely high-quality data and to deeply think and chew over it. The models will work harder on thinking through a small portion of known high-quality data. (Reddit, not verbatim, 22/Jan/2024)
One researcher (22/Jan/2024) similarly notes:
…potentially ‘infinity efficient’ because they may be one-time costs to create. Depending on the details, you may simply create them once and then never again. For example, in ‘AlphaGeometry’, it seems likely that for most problems there’s going to be one and only one best & shortest proof, and that any search process would converge upon it quickly, and now you can just train all future geometry models on that ideal proof. Similarly, in chess or Go I expect that in the overwhelming majority of positions (even excluding the opening book & endgame databases), the best move is known and the engines aren’t going to change the choice no matter how long you run them. ‘Gold datasets’ may be a good moat.
For text training, we’ve now hit massive datasets like the 125TB (30 trillion token) RedPajama-Data-v2, and I continue to track the other highlights on the Datasets Table.
Nearly three years after my data quality paper, are we finally on the way to higher quality (and perhaps temporarily smaller) datasets rather than ‘more is better’?
Explore more in my Mar/2022 comprehensive analysis of datasets, ‘What’s in my AI?’.
2/Jun/2023: OpenAI CEO updates, requested to be removed from the web, archived here.
OpenAI CEO updates Jun/2023
Archived from: https://humanloop.com/blog/openai-plans
1. OpenAI is heavily GPU limited at present
A common theme that came up throughout the discussion was that currently OpenAI is extremely GPU-limited and this is delaying a lot of their short-term plans. The biggest customer complaint was about the reliability and speed of the API. Sam acknowledged their concern and explained that most of the issue was a result of GPU shortages.
The longer 32k context can’t yet be rolled out to more people. OpenAI haven’t overcome the O(n^2) scaling of attention and so whilst it seemed plausible they would have 100k – 1M token context windows soon (this year) anything bigger would require a research breakthrough.
The finetuning API is also currently bottlenecked by GPU availability. They don’t yet use efficient finetuning methods like Adapters or LoRa and so finetuning is very compute-intensive to run and manage. Better support for finetuning will come in the future. They may even host a marketplace of community contributed models.
Dedicated capacity offering is limited by GPU availability. OpenAI also offers dedicated capacity, which provides customers with a private copy of the model. To access this service, customers must be willing to commit to a $100k spend upfront.
2. OpenAI’s near-term roadmap
Sam shared what he saw as OpenAI’s provisional near-term roadmap for the API.
2023:
- Cheaper and faster GPT-4 — This is their top priority. In general, OpenAI’s aim is to drive “the cost of intelligence” down as far as possible and so they will work hard to continue to reduce the cost of the APIs over time.
- Longer context windows — Context windows as high as 1 million tokens are plausible in the near future.
- Finetuning API — The finetuning API will be extended to the latest models but the exact form for this will be shaped by what developers indicate they really want.
- A stateful API — When you call the chat API today, you have to repeatedly pass through the same conversation history and pay for the same tokens again and again. In the future there will be a version of the API that remembers the conversation history.
2024:
- Multimodality — This was demoed as part of the GPT-4 release but can’t be extended to everyone until after more GPUs come online.
3. Plugins “don’t have PMF” and are probably not coming to the API anytime soon
A lot of developers are interested in getting access to ChatGPT plugins via the API but Sam said he didn’t think they’d be released any time soon. The usage of plugins, other than browsing, suggests that they don’t have PMF yet. He suggested that a lot of people thought they wanted their apps to be inside ChatGPT but what they really wanted was ChatGPT in their apps.
4. OpenAI will avoid competing with their customers — other than with ChatGPT
Quite a few developers said they were nervous about building with the OpenAI APIs when OpenAI might end up releasing products that are competitive to them. Sam said that OpenAI would not release more products beyond ChatGPT. He said there was a history of great platform companies having a killer app and that ChatGPT would allow them to make the APIs better by being customers of their own product. The vision for ChatGPT is to be a super smart assistant for work but there will be a lot of other GPT use-cases that OpenAI won’t touch.
5. Regulation is needed but so is open source
While Sam is calling for regulation of future models, he didn’t think existing models were dangerous and thought it would be a big mistake to regulate or ban them. He reiterated his belief in the importance of open source and said that OpenAI was considering open-sourcing GPT-3. Part of the reason they hadn’t open-sourced yet was that he was skeptical of how many individuals and companies would have the capability to host and serve large LLMs.
6. The scaling laws still hold
Recently many articles have claimed that “the age of giant AI Models is already over”. This wasn’t an accurate representation of what was meant.
OpenAI’s internal data suggests the scaling laws for model performance continue to hold and making models larger will continue to yield performance. The rate of scaling can’t be maintained because OpenAI had made models millions of times bigger in just a few years and doing that going forward won’t be sustainable. That doesn’t mean that OpenAI won’t continue to try to make the models bigger, it just means they will likely double or triple in size each year rather than increasing by many orders of magnitude.
The fact that scaling continues to work has significant implications for the timelines of AGI development. The scaling hypothesis is the idea that we may have most of the pieces in place needed to build AGI and that most of the remaining work will be taking existing methods and scaling them up to larger models and bigger datasets. If the era of scaling was over then we should probably expect AGI to be much further away. The fact the scaling laws continue to hold is strongly suggestive of shorter timelines.
29/May/2023: NVIDIA Announces DGX GH200 AI Supercomputer (NVIDIA). ‘New Class of AI Supercomputer Connects 256 Grace Hopper Superchips Into Massive, 1-Exaflop, 144TB GPU for Giant Models… GH200 superchips eliminate the need for a traditional CPU-to-GPU PCIe connection by combining an Arm-based NVIDIA Grace™ CPU with an NVIDIA H100 Tensor Core GPU in the same package, using NVIDIA NVLink-C2C chip interconnects.’
Expect trillion-parameter models like OpenAI GPT-5, Anthropic Claude-Next, and beyond to be trained with this groundbreaking hardware. Some have estimated that this could train language models up to 80 trillion parameters, which gets us closer to brain-scale.
20/May/2023: Updated GPT-4 chart for reference.
By request, here’s a simplified version of this full GPT-4 vs human viz; easier to read on a big screen!
Download source (PDF)
19/May/2023: OpenAI CEO (Elevate):
…with the arrival of GPT-4, people started building entire companies around it. I believe that GPT-5, 6, and 7 will continue this trajectory in future years, really increasing the utility they can provide.
This development is a big, new, exciting thing to have in the world. It’s as though all of computing got an upgrade.
I think we’ll look back at this period like we look back at the period where people were discovering fundamental physics. The fact that we’re discovering how to predict the intelligence of a trained AI before we start training it suggests that there is something close to a natural law here. We can predictably say this much compute, this big of a neural network, this training data – these will determine the capabilities of the model. Now we can predict how it’ll score on some tests.
…whether we can predict the sort of qualitative new things – the new capabilities that didn’t exist at all in GPT-4 but do exist in future versions like GPT-5. That seems important to figure out. But right now, we can say, ‘Here’s how we predict it’ll do on this evaluation or this metric.’ I really do think we’ll look back at this period as if we were all living through one of the most important periods of human discovery.
I believe that this will be a monumental deal in terms of how we think about when we go beyond human intelligence. However, I don’t think that’s quite the right framework because it’ll happen in some areas and not others. Already, these systems are superhuman in some limited areas and extremely bad in others, and I think that’s fine.
…this analogy: it’s like everybody’s going to be the CEO of all of the work they want to do. They’ll have tons of people that they’re able to coordinate and direct, provide the text and the feedback on. But they’ll also have lots of agents, for lack of a better word, that go off and do increasingly complex tasks.
16/May/2023: OpenAI CEO to Congress: ‘We are not currently training what will be GPT-5; we don’t have plans to do it in the next 6 months [to 16/Nov/2023]’.
"We are not currently training what will be GPT-5; we don't have plans to do it in the next 6 months"
– Sam Altman, under oath— Daniel Eth (yes, Eth is my actual last name) (@daniel_271828) May 16, 2023
11/May/2023: Microsoft Korea: ‘We are preparing for GPT-5, and GPT-6 will also be released.’ (Yonhap News Agency (Korean)).
13/Feb/2023: Morgan Stanley research note:
We think that GPT 5 is currently being trained on 25k GPUs – $225 mm or so of NVIDIA hardware…
The current version of the model, GPT-5, will be trained in the same facility—announced in 2020 [May/2020, Microsoft], the supercomputer designed specifically for OpenAI has 285k CPU cores, 10k GPU cards, and 400 Gb/s connectivity for each GPU server; our understanding is that there has been substantial expansion since then. From our conversation, GPT-5 is being trained on about 25k GPUs, mostly A100s, and it takes multiple months; that’s about $225m of NVIDIA hardware, but importantly this is not the only use, and many of the same GPUs were used to train GPT-3 and GPT-4…
We also would expect the number of large language models under development to remain relatively small. IF the training hardware for GPT-5 is $225m worth of NVIDIA hardware, that’s close to $1b of overall hardware investment; that isn’t something that will be undertaken lightly. We see large language models at a similar scale being developed at every hyperscaler, and at multiple startups.
Morgan Stanley on Nvidia’s opportunity with ChatGPT etc 👇🏻
“We think that GPT 5 is currently being trained on 25k GPUs – $225 mm or so of NVIDIA hardware…”
Let’s hope @annerajb and @GroggyTBear have sourced enough GPUs for ‘23. We’re pretty much sold out. Sorry 😔😔😔$NVDA pic.twitter.com/k6X9YOSsgF
— David Tayar (@davidtayar5) February 13, 2023
Remainder of note 👇🏻 pic.twitter.com/TSKeYqetNP
— David Tayar (@davidtayar5) February 20, 2023
Datacenter location
Models Table
Summary of current models: View the full data (Google sheets)Dataset
A Comprehensive Analysis of Datasets Likely Used to Train GPT-5
Alan D. Thompson
LifeArchitect.ai
August 2024
27 pages incl title page, references, appendices.
Timeline to GPT-6
Date | Milestone |
11/Jun/2018 | GPT-1 announced on the OpenAI blog. |
14/Feb/2019 | GPT-2 announced on the OpenAI blog. |
28/May/2020 | Initial GPT-3 preprint paper published to arXiv. |
11/Jun/2020 | GPT-3 API private beta. |
22/Sep/2020 | GPT-3 licensed to Microsoft. |
18/Nov/2021 | GPT-3 API opened to the public. |
27/Jan/2022 | InstructGPT released as text-davinci-002, now known as GPT-3.5. InstructGPT preprint paper Mar/2022. |
28/Jul/2022 | Exploring data-optimal models with FIM, paper on arXiv. |
1/Sep/2022 | GPT-3 model pricing cut by 66% for davinci model. |
21/Sep/2022 | Whisper (speech recognition) announced on the OpenAI blog. |
28/Nov/2022 | GPT-3.5 expanded to text-davinci-003, announced via email: 1. Higher quality writing. 2. Handles more complex instructions. 3. Better at longer form content generation. |
30/Nov/2022 | ChatGPT announced on the OpenAI blog. |
14/Mar/2023 | GPT-4 released. |
31/May/2023 | GPT-4 MathMix and step by step, paper on arXiv. |
6/Jul/2023 | GPT-4 available via API. |
25/Sep/2023 | GPT-4V finally released. |
13/May/2024 | GPT-4o announced. |
18/Jul/2024 | GPT-4o mini announced. |
12/Sep/2024 | o1 released. |
2024 | GPT-5… |
2025 | GPT-6… |
AI Race
Download source (PDF)
Permissions: Yes, you can use these visualizations anywhere, please leave the citation intact.
Video
OpenAI’s diplomatic mission (2023)
On 9/Jun/2023, at a fireside chat in Seoul, Korea, the OpenAI CEO acknowledged he was on a “diplomatic mission.” After the release of GPT-4 in Mar/2023, OpenAI staff visited the following regions:
- Canada: Toronto
- USA: Washington D.C.
- Brazil: Rio De Janeiro
- Nigeria: Lagos
- Spain: Madrid (the Spanish Presidency of the Council of the European Union ran from 1/July/2023-31/Dec/2023)
- Belgium: Brussels
- Germany: Munich
- UK: London
- France: Paris
- Israel: Tel Aviv
- UAE: Dubai
- India: New Delhi
- Singapore
- Indonesia: Jakarta
- South Korea: Seoul
- Japan: Tokyo
- Australia: Melbourne
visiting toronto, DC, rio, lagos, madrid, brussels, munich, london, paris, tel aviv, dubai, new delhi, singapore, jakarta, seoul, tokyo, melbourne.
also hoping to give talks in some of the cities and meet with policymakers.
— Sam Altman (@sama) March 29, 2023
Plus:
- Jordan
- Qatar
- China: Beijing
- Poland
Sources:
https://foreignpolicy.com/2023/06/20/openai-ceo-diplomacy-artificial-intelligence/
https://twitter.com/sama/status/1665320086415060992
https://techcrunch.com/2023/05/25/sam-altman-european-tour/
AGI
Read more about Alan’s conservative countdown to AGI…
Get The Memo
by Dr Alan D. Thompson · Be inside the lightning-fast AI revolution.Informs research at Apple, Google, Microsoft · Bestseller in 142 countries.
Artificial intelligence that matters, as it happens, in plain English.
Get The Memo.
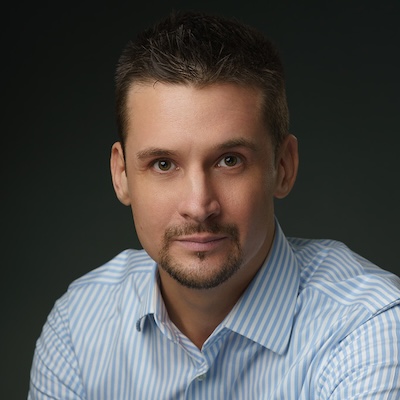
This page last updated: 18/Oct/2024. https://lifearchitect.ai/gpt-6/↑