TL;DR: I will no longer be addressing criticisms regarding AI directly. It would be a misuse of your imagination, and a misuse of my time. Happily, there are other people who have devoted significant time to addressing concerns about AI. What follows is an extract from a 20,000-word chapter published by Dr Ray Kurzweil in 2005. Ray has devoted his life to the study and prediction of advances in AI technology.
Humans are prone to fall victim to a concept called negativity bias (Wikipedia).
We no longer live in the days of the saber-toothed tiger, so this kind of amygdala response to danger is unnecessary, and to be quite frank, both cheap and lazy. Anyone can criticise. Anyone can say ‘yeh, nah, that’s bullshit.’ It takes courage and power to face the right direction, and to seek out the solutions, rather than the problems.
You can’t stop the river of advances.
These ethical debates are like stones in a stream. The water runs around them. You haven’t seen any of these… technologies held up for one week by any of these debates.
— Dr Ray Kurzweil (January 2020)
I acknowledge Dr Ray Kurzweil for his impactful book, The Singularity is Near, available via Amazon. The content below is copyrighted material provided under fair use (less than one full chapter).
— Alan
- I don’t believe it (The Criticism from Incredulity)
- It will flatten (The Criticism from Malthus)
- Software will be a bottleneck (The Criticism from Software), not shown
- The Criticism from Analog Processing, not shown
- We don’t understand the brain (The Criticism from the Complexity of Neural Processing)
- The Criticism from Microtubules and Quantum Computing, not shown
- Some problems are unsolvable by computers (The Criticism from the Church-Turing Thesis)
- Machines have high failure rates (The Criticism from Failure Rates)
- The Criticism from “Lock-In”, not shown
- AI isn’t conscious (The Criticism from Ontology: Can a Computer Be Conscious?)
- The distribution of AI won’t be equal (The Criticism from the Rich-Poor Divide)
- Governments will slow AI down (The Criticism from the Likelihood of Government Regulation)
- AI isn’t spiritual (The Criticism from Theism)
- AI isn’t biological (The Criticism from Holism)
CHAPTER NINE
Response to Critics
The human mind likes a strange idea as little as the body likes a strange protein and resists it with a similar energy.
—W. I. Beveridge
If a … scientist says that something is possible he is almost certainly right, but if he says that it is impossible he is very probably wrong.
—Arthur C. Clarke
A Panoply of Criticisms
In The Age of Spiritual Machines, I began to examine some of the accelerating trends that I have sought to explore in greater depth in this book. ASM inspired a broad variety of reactions, including extensive discussions of the profound, imminent changes it considered (for example, the promise-versus-peril debate prompted by Bill Joy’s Wired story, “Why the Future Doesn’t Need Us,” as I reviewed in the previous chapter). The response also included attempts to argue on many levels why such transformative changes would not, could not, or should not happen. Here is a summary of the critiques I will be responding to in this chapter:
- The “criticism from Malthus”: It’s a mistake to extrapolate exponential trends indefinitely, since they inevitably run out of resources to maintain the exponential growth. Moreover, we won’t have enough energy to power the extraordinarily dense computational platforms forecast, and even if we did they would be as hot as the sun. Exponential trends do reach an asymptote, but the matter and energy resources needed for computation and communication are so small per compute and per bit that these trends can continue to the point where nonbiological intelligence is trillions of trillions of times more powerful than biological intelligence. Reversible computing can reduce energy requirements, as well as heat dissipation, by many orders of magnitude. Even restricting computation to “cold” computers will achieve nonbiological computing platforms that vastly outperform biological intelligence.
- The “criticism from software”: We’re making exponential gains in hardware, but software is stuck in the mud. Although the doubling time for progress in software is longer than that for computational hardware, software is also accelerating in effectiveness, efficiency, and complexity. Many software applications, ranging from search engines to games, routinely use AI techniques that were only research projects a decade ago. Substantial gains have also been made in the overall complexity of software, in software productivity, and in the efficiency of software in solving key algorithmic problems. Moreover, we have an effective game plan to achieve the capabilities of human intelligence in a machine: reverse engineering the brain to capture its principles of operation and then implementing those principles in brain-capable computing platforms. Every aspect of brain reverse engineering is accelerating: the spatial and temporal resolution of brain scanning, knowledge about every level of the brain’s operation, and efforts to realistically model and simulate neurons and brain regions.
- The “criticism from analog processing”: Digital computation is too rigid because digital bits are either on or off. Biological intelligence is mostly analog, so subtle gradations can be considered. It’s true that the human brain uses digital-controlled analog methods, but we can also use such methods in our machines. Moreover, digital computation can simulate analog transactions to any desired level of accuracy, whereas the converse statement is not true.
- The “criticism from the complexity of neural processing”: The information processes in the interneuronal connections (axons, dendrites, synapses) are far more complex than the simplistic models used in neural nets. True, but brain-region simulations don’t use such simplified models. We have achieved realistic mathematical models and computer simulations of neurons and interneuronal connections that do capture the nonlinearities and intricacies of their biological counterparts. Moreover, we have found that the complexity of processing brain regions is often simpler than the neurons they comprise. We already have effective models and simulations for several dozen regions of the human brain. The genome contains only about thirty to one hundred million bytes of design information when redundancy is considered, so the design information for the brain is of a manageable level.
- The “criticism from microtubules and quantum computing”: The microtubules in neurons are capable of quantum computing, and such quantum computing is a prerequisite for consciousness. To “upload” a personality, one would have to capture its precise quantum state. No evidence exists to support either of these statements. Even if true, there is nothing that bars quantum computing from being carried out in nonbiological systems. We routinely use quantum effects in semiconductors (tunneling in transistors, for example), and machine-based quantum computing is also progressing. As for capturing a precise quantum state, I’m in a very different quantum state than I was before writing this sentence. So am I already a different person? Perhaps I am, but if one captured my state a minute ago, an upload based on that information would still successfully pass a “Ray Kurzweil” Turing test.
- The “criticism from the Church-Turing thesis”: We can show that there are broad classes of problems that cannot be solved by any Turing machine. It can also be shown that Turing machines can emulate any possible computer (that is, there exists a Turing machine that can solve any problem that any computer can solve), so this demonstrates a clear limitation on the problems that a computer can solve. Yet humans are capable of solving these problems, so machines will never emulate human intelligence. Humans are no more capable of universally solving such “unsolvable” problems than machines. Humans can make educated guesses to solutions in certain instances, but machines can do the same thing and can often do so more quickly.
- The “criticism from failure rates”: Computer systems are showing alarming rates of catastrophic failure as their complexity increases. Thomas Ray writes that we are “pushing the limits of what we can effectively design and build through conventional approaches.” We have developed increasingly complex systems to manage a broad variety of mission-critical tasks, and failure rates in these systems are very low. However, imperfection is an inherent feature of any complex process, and that certainly includes human intelligence.
- The “criticism from ‘lock-in’ “: The pervasive and complex support systems (and the huge investments in these systems) required by such fields as energy and transportation are blocking innovation, so this will prevent the kind of rapid change envisioned for the technologies underlying the Singularity. It is specifically information processes that are growing exponentially in capability and price-performance. We have already seen rapid paradigm shifts in every aspect of information technology, unimpeded by any lock-in phenomenon (despite large infrastructure investments in such areas as the Internet and telecommunications). Even the energy and transportation sectors will witness revolutionary changes from new nanotechnology-based innovations.
- The “criticism from ontology”: John Searle describes several versions of his Chinese Room analogy. In one formulation a man follows a written program to answer questions in Chinese. The man appears to be answering questions competently in Chinese, but since he is “just mechanically following a written program, he has no real understanding of Chinese and no real awareness of what he is doing. The “man” in the room doesn’t understand anything, because, after all, “he is just a computer,” according to Searle. So clearly, computers cannot understand what they are doing, since they are just following rules. Searle’s Chinese Room arguments are fundamentally tautological, as they just assume his conclusion that computers cannot possibly have any real understanding. Part of the philosophical sleight of hand in Searle’s simple analogies is a matter of scale. He purports to describe a simple system and then asks the reader to consider how such a system could possibly have any real understanding. But the characterization itself is misleading. To be consistent with Searle’s own assumptions the Chinese Room system that Searle describes would have to be as complex as a human brain and would, therefore, have as much understanding as a human brain. The man in the analogy would be acting as the central-processing unit, only a small part of the system. While the man may not see it, the understanding is distributed across the entire pattern of the program itself and the billions of notes he would have to make to follow the program. Consider that I understand English, but none of my neurons do. My understanding is represented in vast patterns of neurotransmitter strengths, synaptic clefts, and interneuronal connections.
- The “criticism from the rich-poor divide”: It’s likely that through these technologies the rich may obtain certain opportunities that the rest of humankind does not have access to. This, of course, would be nothing new, but I would point out that because of the ongoing exponential growth of price-performance, all of these technologies quickly become so inexpensive as to become almost free.
- The “criticism from the likelihood of government regulation”: Governmental regulation will slow down and stop the acceleration of technology. Although the obstructive potential of regulation is an important concern, it has had as of yet little measurable effect on the trends discussed in this book. Absent a worldwide totalitarian state, the economic and other forces underlying technical progress will only grow with ongoing advances. Even controversial issues such as stem-cell research end up being like stones in a stream, the flow of progress rushing around them .
- The “criticism from theism”: According to William A. Dembski, “contemporary materialists such as Ray Kurzweil … see the motions and modifications of matter as sufficient to account for human mentality.” But materialism is predictable, whereas reality is not. Predictability [is] materialism’s main virtue … and hollowness [is] its main fault.” Complex systems of matter and energy are not predictable, since they are based on a vast number of unpredictable quantum events. Even if we accept a “hidden variables” interpretation of quantum mechanics (which says that quantum events only appear to be unpredictable but are based on undetectable hidden variables), the behavior of a complex system would still be unpredictable in practice. All of the trends show that we are clearly headed for nonbiological systems that are as complex as their biological counterparts. Such future systems will be no more “hollow” than humans and in many cases will be based on the reverse engineering of human intelligence. We don’t need to go beyond the capabilities of patterns of matter and energy to account for the capabilities of human intelligence .
- The “criticism from holism”: To quote Michael Denton, organisms are “self-organizing, … self-referential, … self-replicating, … reciprocal, … self-formative, and … holistic.” Such organic forms can be created only through biological processes, and such forms are “immutable, … impenetrable, and … fundamental realities of existence.”1 It’s true that biological design represents a profound set of principles. However, machines can use—and already are using—these same principles, and there is nothing that restricts nonbiological systems from harnessing the emergent properties of the patterns found in the biological world.
I’ve engaged in countless debates and dialogues responding to these challenges in a diverse variety of forums. One of my goals for this book is to provide a comprehensive response to the most important criticisms I have encountered. Most of my rejoinders to these critiques on feasibility and inevitability have been discussed throughout this book, but in this chapter I want to offer a detailed reply to several of the more interesting ones.
The Criticism from Incredulity
Perhaps the most candid criticism of the future I have envisioned here is simple disbelief that such profound changes could possibly occur. Chemist Richard Smalley, for example, dismisses the idea of nanobots being capable of performing missions in the human bloodstream as just “silly.” But scientists’ ethics call for caution in assessing the prospects for current work, and such reasonable prudence unfortunately often leads scientists to shy away from considering the power of generations of science and technology far beyond today’s frontier. With the rate of paradigm shift occurring ever more quickly, this ingrained pessimism does not serve society’s needs in assessing scientific capabilities in the decades ahead. Consider how incredible today’s technology would seem to people even a century ago.
A related criticism is based on the notion that it is difficult to predict the future, and any number of bad predictions from other futurists in earlier eras can be cited to support this. Predicting which company or product will succeed is indeed very difficult, if not impossible. The same difficulty occurs in predicting which technical design or standard will prevail. (For example, how will the wireless-communication protocols WiMAX, CDMA, and 3G fare over the next several years?) However, as this book has extensively argued, we find remarkably precise and predictable exponential trends when assessing the overall effectiveness (as measured by price-performance, bandwidth, and other measures of capability) of information technologies. For example, the smooth exponential growth of the price-performance of computing dates back over a century. Given that the minimum amount of matter and energy required to compute or transmit a bit of information is known to be vanishingly small, we can confidently predict the continuation of these information-technology trends at least through this next century. Moreover, we can reliably predict the capabilities of these technologies at future points in time.
Consider that predicting the path of a single molecule in a gas is essentially impossible, but predicting certain properties of the entire gas (composed of a great many chaotically interacting molecules) can reliably be predicted through the laws of thermodynamics. Analogously, it is not possible to reliably predict the results of a specific project or company, but the overall capabilities of information technology (comprised of many chaotic activities) can nonetheless be dependably anticipated through the law of accelerating returns.
Many of the furious attempts to argue why machines—nonbiological systems—cannot ever possibly compare to humans appear to be fueled by this basic reaction of incredulity. The history of human thought is marked by many attempts to refuse to accept ideas that seem to threaten the accepted view that our species is special. Copernicus’s insight that the Earth was not at the center of the universe was resisted, as was Darwin’s that we were only slightly evolved from other primates. The notion that machines could match and even exceed human intelligence appears to challenge human status once again.
In my view there is something essentially special, after all, about human beings. We were the first species on Earth to combine a cognitive function and an effective opposable appendage (the thumb), so we were able to create technology that would extend our own horizons. No other species on Earth has accomplished this. (To be precise, we’re the only surviving species in this ecological niche—others, such as the Neanderthals, did not survive.) And as I discussed in chapter 6, we have yet to discover any other such civilization in the universe.
The Criticism from Malthus
Exponential Trends Don’t Last Forever. The classical metaphorical example of exponential trends hitting a wall is known as “rabbits in Australia.” A species happening upon a hospitable new habitat will expand its numbers exponentially until its growth hits the limits of the ability of that environment to support it. Approaching this limit to exponential growth may even cause an overall reduction in numbers—for example, humans noticing a spreading pest may seek to eradicate it. Another common example is a microbe that may grow exponentially in an animal body until a limit is reached: the ability of that body to support it, the response of its immune system, or the death of the host.
Even the human population is now approaching a limit. Families in the more developed nations have mastered means of birth control and have set relatively high standards for the resources they wish to provide their children. As a result population expansion in the developed world has largely stopped. Meanwhile people in some (but not all) underdeveloped countries have continued to seek large families as a means of social security, hoping that at least one child will survive long enough to support them in old age. However, with the law of accelerating returns providing more widespread economic gains, the overall growth in human population is slowing.
So isn’t there a comparable limit to the exponential trends that we are witnessing for information technologies?
The answer is yes, but not before the profound transformations described throughout this book take place. As I discussed in chapter 3, the amount of matter and energy required to compute or transmit one bit is vanishingly small. By using reversible logic gates, the input of energy is required only to transmit results and to correct errors. Otherwise, the heat released from each computation is immediately recycled to fuel the next computation.
As I discussed in chapter 5, nanotechnology-based designs for virtually all applications—computation, communication, manufacturing, and transportation—will require substantially less energy than they do today. Nanotechnology will also facilitate capturing renewable energy sources such as sunlight. We could meet all of our projected energy needs of thirty trillion watts in 2030 with solar power if we captured only 0.03 percent (three ten-thousandths) of the sun’s energy as it hit the Earth. This will be feasible with extremely inexpensive, lightweight, and efficient nanoengineered solar panels together with nano-fuel cells to store and distribute the captured energy.
A Virtually Unlimited Limit. As I discussed in chapter 3 an optimally organized 2.2-pound computer using reversible logic gates has about 1025 atoms and can store about 1027 bits. Just considering electromagnetic interactions between the particles, there are at least 1015 state changes per bit per second that can be harnessed for computation, resulting in about 1042 calculations per second in the ultimate “cold” 2.2-pound computer. This is about 1016 times more powerful than all biological brains today. If we allow our ultimate computer to get hot, we can increase this further by as much as 108-fold. And we obviously won’t restrict our computational resources to one kilogram of matter but will ultimately deploy a significant fraction of the matter and energy on the Earth and in the solar system and then spread out from there.
Specific paradigms do reach limits. We expect that Moore’s Law (concerning the shrinking of the size of transistors on a flat integrated circuit) will hit a limit over the next two decades. The date for the demise of Moore’s Law keeps getting pushed back. The first estimates predicted 2002, but now Intel says it won’t take place until 2022. But as I discussed in chapter 2, every time a specific computing paradigm was seen to approach its limit, research interest and pressure increased to create the next paradigm. This has already happened four times in the century-long history of exponential growth in computation (from electromagnetic calculators to relay-based computers to vacuum tubes to discrete transistors to integrated circuits). We have already achieved many important milestones toward the next (sixth) paradigm of computing: three-dimensional self-organizing circuits at the molecular level. So the impending end of a given paradigm does not represent a true limit.
There are limits to the power of information technology, but these limits are vast. I estimated the capacity of the matter and energy in our solar system to support computation to be at least 1070 cps (see chapter 6). Given that there are at least 1020 stars in the universe, we get about 1090 cps for it, which matches Seth Lloyd’s independent analysis. So yes, there are limits, but they’re not very limiting.
The Criticism from Software
[Not shown]
The Criticism from Analog Processing
[Not shown]The Criticism from the Complexity of Neural Processing
Another common criticism is that the fine detail of the brain’s biological design is simply too complex to be modeled and simulated using nonbiological technology. For example, Thomas Ray writes:
The structure and function of the brain or its components cannot be separated. The circulatory system provides life support for the brain, but it also delivers hormones that are an integral part of the chemical information processing function of the brain. The membrane of a neuron is a structural feature defining the limits and integrity of a neuron, but it is also the surface along which depolarization propagates signals. The structural and life-support functions cannot be separated from the handling of information.17
Ray goes on to describe several of the “broad spectrum of chemical communication mechanisms” that the brain exhibits.
In fact, all of these features can readily be modeled, and a great deal of progress has already been made in this endeavor. The intermediate language is mathematics, and translating the mathematical models into equivalent nonbiological mechanisms (examples include computer simulations and circuits using transistors in their native analog mode) is a relatively straightforward process. The delivery of hormones by the circulatory system, for example, is an extremely low-bandwidth phenomenon, which is not difficult to model and replicate. The blood levels of specific hormones and other chemicals influence parameter levels that affect a great many synapses simultaneously.
Thomas Ray concludes that “a metallic computation system operates on fundamentally different dynamic properties and could never precisely and exactly ‘copy’ the function of a brain.” Following closely the progress in the related fields of neurobiology, brain scanning, neuron and neural-region modeling, neuron-electronic communication, neural implants, and related endeavors, we find that our ability to replicate the salient functionality of biological information processing can meet any desired level of precision. In other words the copied functionality can be “close enough” for any conceivable purpose or goal, including satisfying a Turing-test judge. Moreover, we find that efficient implementations of the mathematical models require substantially less computational capacity than the theoretical potential of the biological neuron clusters being modeled. In chapter 4, I reviewed a number of brain-region models (Watts’s auditory regions, the cerebellum, and others) that demonstrate this.
Brain Complexity. Thomas Ray also makes the point that we might have difficulty creating a system equivalent to “billions of lines of code,” which is the level of complexity he attributes to the human brain. This figure, however, is highly inflated, for as we have seen our brains are created from a genome of only about thirty to one hundred million bytes of unique information (eight hundred million bytes without compression, but compression is clearly feasible given the massive redundancy), of which perhaps two thirds describe the principles of operation of the brain. It is self-organizing processes that incorporate significant elements of randomness (as well as exposure to the real world) that enable so relatively small an amount of design information to be expanded to the thousands of trillions of bytes of information represented in a mature human brain. Similarly, the task of creating human-level intelligence in a nonbiological entity will involve creating not a massive expert system comprising billions of rules or lines of code but rather a learning, chaotic, self-organizing system, one that is ultimately biologically inspired.
Ray goes on to write, “The engineers among us might propose nanomolecular devices with fullerene switches, or even DNA-like computers. But I am sure they would never think of neurons. Neurons are astronomically large structures compared to the molecules we are starting with.”
This is exactly my own point. The purpose of reverse engineering the human brain is not to copy the digestive or other unwieldy processes of biological neurons but rather to understand their key information-processing methods. The feasibility of doing this has already been demonstrated in dozens of contemporary projects. The complexity of the neuron clusters being emulated is scaling up by orders of magnitude, along with all of our other technological capabilities.
A Computer’s Inherent Dualism. Neuroscientist Anthony Bell of Redwood Neuroscience Institute articulates two challenges to our ability to model and simulate the brain with computation. In the first he maintains that
a computer is an intrinsically dualistic entity, with its physical set-up designed not to interfere with its logical set-up, which executes the computation. In empirical investigation, we find that the brain is not a dualistic entity. Computer and program may be two, but mind and brain are one. The brain is thus not a machine, meaning it is not a finite model (or computer) instantiated physically in such a way that the physical instantiation does not interfere with the execution of the model (or program).18
This argument is easily dispensed with. The ability to separate in a computer the program from the physical instantiation that performs the computation is an advantage, not a limitation. First of all, we do have electronic devices with dedicated circuitry in which the “computer and program” are not two, but one. Such devices are not programmable but are hardwired for one specific set of algorithms. Note that I am not just referring to computers with software (called “firmware”) in read-only memory, as may be found in a cell phone or pocket computer. In such a system, the electronics and the software may still be considered dualistic even if the program cannot easily be modified.
I am referring instead to systems with dedicated logic that cannot be programmed at all—such as application-specific integrated circuits (used, for example, for image and signal processing). There is a cost efficiency in implementing algorithms in this way, and many electronic consumer products use such circuitry. Programmable computers cost more but provide the flexibility of allowing the software to be changed and upgraded. Programmable computers can emulate the functionality of any dedicated system, including the algorithms that we are discovering (through the efforts to reverse engineer the brain) for neural components, neurons, and brain regions.
There is no validity to calling a system in which the logical algorithm is inherently tied to its physical design “not a machine.” If its principles of operation can be understood, modeled in mathematical terms, and then instantiated on another system (whether that other system is a machine with unchangeable dedicated logic or software on a programmable computer), then we can consider it to be a machine and certainly an entity whose capabilities can be re-created in a machine. As I discussed extensively in chapter 4, there are no barriers to our discovering the brain’s principles of operation and successfully modeling and simulating them, from its molecular interactions upward.
Bell refers to a computer’s “physical set-up [that is] designed not to interfere with its logical set-up,” implying that the brain does not have this “limitation.” He is correct that our thoughts do help create our brains, and as I pointed out earlier we can observe this phenomenon in dynamic brain scans. But we can readily model and simulate both the physical and logical aspects of the brain’s plasticity in software. The fact that software in a computer is separate from its physical instantiation is an architectural advantage in that it allows the same software to be applied to ever-improving hardware. Computer software, like the brain’s changing circuits, can also modify itself, as well as be upgraded.
Computer hardware can likewise be upgraded without requiring a change in software. It is the brain’s relatively fixed architecture that is severely limited. Although the brain is able to create new connections and neurotransmitter patterns, it is restricted to chemical signaling more than one million times slower than electronics, to the limited number of interneuronal connections that can fit inside our skulls, and to having no ability to be upgraded, other than through the merger with nonbiological intelligence that I’ve been discussing.
Levels and Loops. Bell also comments on the apparent complexity of the brain:
Molecular and biophysical processes control the sensitivity of neurons to incoming spikes (both synaptic efficiency and post-synaptic responsivity), the excitability of the neuron to produce spikes, the patterns of spikes it can produce and the likelihood of new synapses forming (dynamic rewiring), to list only four of the most obvious interferences from the subneural level. Furthermore, transneural volume effects such as local electric fields and the transmembrane diffusion of nitric oxide have been seen to influence, respectively, coherent neural firing, and the delivery of energy (blood flow) to cells, the latter of which directly correlates with neural activity.
The list could go on. I believe that anyone who seriously studies neuromodulators, ion channels or synaptic mechanism and is honest, would have to reject the neuron level as a separate computing level, even while finding it to be a useful descriptive level.19
Although Bell makes the point here that the neuron is not the appropriate level at which to simulate the brain, his primary argument here is similar to that of Thomas Ray above: the brain is more complicated than simple logic gates.
He makes this explicit:
To argue that one piece of structured water or one quantum coherence is a necessary detail in the functional description of the brain would clearly be ludicrous. But if, in every cell, molecules derive systematic functionality from these submolecular processes, if these processes are used all the time, allover the brain, to reflect, record and propagate spatiotemporal correlations of molecular fluctuations, to enhance or diminish the probabilities and specificities of reactions, then we have a situation qualitatively different from the logic gate.
At one level he is disputing the simplistic models of neurons and interneuronal connections used in many neural-net projects. Brain-region simulations don’t use these simplified models, however, but rather apply realistic mathematical models based on the results from brain reverse engineering.
The real point that Bell is making is that the brain is immensely complicated, with the consequent implication that it will therefore be very difficult to understand, model, and simulate its functionality. The primary problem with Bell’s perspective is that he fails to account for the self-organizing, chaotic, and fractal nature of the brain’s design. It’s certainly true that the brain is complex, but a lot of the complication is more apparent than real. In other words, the principles of the design of the brain are simpler than they appear.
To understand this, let’s first consider the fractal nature of the brain’s organization, which I discussed in chapter 2. A fractal is a rule that is iteratively applied to create a pattern or design. The rule is often quite simple, but because of the iteration the resulting design can be remarkably complex. A famous example of this is the Mandelbrot set devised by mathematician Benoit Mandelbrot.20 Visual images of the Mandelbrot set are remarkably complex, with endlessly complicated designs within designs. As we look at finer and finer detail in an image of the Mandelbrot set, the complexity never goes away, and we continue to see ever finer complication. Yet the formula underlying all of this complexity is amazingly simple: the Mandelbrot set is characterized by a single formula Z = Z2 + C, in which Z is a “complex” (meaning two-dimensional) number and C is a constant. The formula is iteratively applied, and the resulting two-dimensional points are graphed to create the pattern.
[image not shown]
The point here is that a simple design rule can create a lot of apparent complexity. Stephen Wolfram makes a similar point using simple rules on cellular automata (see chapter 2). This insight holds true for the brain’s design. As I’ve discussed, the compressed genome is a relatively compact design, smaller than some contemporary software programs. As Bell points out, the actual implementation of the brain appears far more complex than this. Just as with the Mandelbrot set, as we look at finer and finer features of the brain, we continue to see apparent complexity at each level. At a macro level the pattern of connections looks complicated, and at a micro level so does the design of a single portion of a neuron such as a dendrite. I’ve mentioned that it would take at least thousands of trillions of bytes to characterize the state of a human brain, but the design is only tens of millions of bytes. So the ratio of the apparent complexity of the brain to the design information is at least one hundred million to one. The brain’s information starts out as largely random information, but as the brain interacts with a complex environment (that is, as the person learns and matures), that information becomes meaningful.
The actual design complexity is governed by the compressed information in the design (that is, the genome and supporting molecules), not by the patterns created through the iterative application of the design rules. I would agree that the roughly thirty to one hundred million bytes of information in the genome do not represent a simple design (certainly far more complex than the six characters in the definition of the Mandelbrot set), but it is a level of complexity that we can already manage with our technology. Many observers are confused by the apparent complexity in the brain’s physical instantiation, failing to recognize that the fractal nature of the design means that the actual design information is far simpler than what we see in the brain.
I also mentioned in chapter 2 that the design information in the genome is a probabilistic fractal, meaning that the rules are applied with a certain amount of randomness each time a rule is iterated. There is, for example, very little information in the genome describing the wiring pattern for the cerebellum, which comprises more than half the neurons in the brain. A small number of genes describe the basic pattern of the four cell types in the cerebellum and then say in essence, “Repeat this pattern several billion times with some random variation in each repetition.” The result may look very complicated, but the design information is relatively compact.
Bell is correct that trying to compare the brain’s design to a conventional computer would be frustrating. The brain does not follow a typical top-down (modular) design. It uses its probabilistic fractal type of organization to create processes that are chaotic—that is, not fully predictable. There is a well-developed body of mathematics devoted to modeling and simulating chaotic systems, which are used to understand phenomena such as weather patterns and financial markets, that is also applicable to the brain.
Bell makes no mention of this approach. He argues why the brain is dramatically different from conventional logic gates and conventional software design, which leads to his unwarranted conclusion that the brain is not a machine and cannot be modeled by a machine. While he is correct that standard logic gates and the organization of conventional modular software are not the appropriate way to think about the brain, that does not mean that we are unable to simulate the brain on a computer. Because we can describe the brain’s principles of operation in mathematical terms, and since we can model any mathematical process (including chaotic ones) on a computer, we are able to implement these types of simulations. Indeed, we’re making solid and accelerating progress in doing so.
Despite his skepticism Bell expresses cautious confidence that we will understand our biology and brains well enough to improve on them. He writes: “Will there be a transhuman age? For this there is a strong biological precedent in the two major steps in biological evolution. The first, the incorporation into eukaryotic bacteria of prokaryotic symbiotes, and the second, the emergence of multicellular life-forms from colonies of eukaryotes….I believe that something like [a transhumanist age] may happen.”
The Criticism from Microtubules and Quantum Computing
[Not shown]
The Criticism from the Church-Turing Thesis
Early in the twentieth century mathematicians Alfred North Whitehead and Bertrand Russell published their seminal work, Principia Mathematica, which sought to determine axioms that could serve as the basis for all of mathematics.26 However, they were unable to prove conclusively that an axiomatic system that can generate the natural numbers (the positive integers or counting numbers) would not give rise to contradictions. It was assumed that such a proof would be found sooner or later, but in the 1930s a young Czech mathematician, Kurt Gödel, stunned the mathematical world by proving that within such a system there inevitably exist propositions that can be neither proved nor disproved. It was later shown that such unprovable propositions are as common as provable ones. Gödel’s incompleteness theorem, which is fundamentally a proof demonstrating that there are definite limits to what logic, mathematics, and by extension computation can do, has been called the most important in all mathematics, and its implications are still being debated.27
A similar conclusion was reached by Alan Turing in the context of understanding the nature of computation. When in 1936 Turing presented the Turing machine (described in chapter 2) as a theoretical model of a computer, which continues today to form the basis of modern computational theory, he reported an unexpected discovery similar to Gödel’s.28 In his paper that year he described the concept of unsolvable problems—that is, problems that are well defined, with unique answers that can be shown to exist, but that we can also show can never be computed by a Turing machine.
The fact that there are problems that cannot be solved by this particular theoretical machine may not seem particularly startling until you consider the other conclusion of Turing’s paper: that the Turing machine can model any computational process. Turing showed that there are as many unsolvable problems as solvable ones, the number of each being the lowest order of infinity, the so-called countable infinity (that is, counting the number of integers). Turing also demonstrated that the problem of determining the truth or falsity of any logical proposition in an arbitrary system of logic powerful enough to represent the natural numbers was one example of an unsolved problem, a result similar to Gödel’s. (In other words, there is no procedure guaranteed to answer this question for all such propositions.)
Around the same time Alonzo Church, an American mathematician and philosopher, published a theorem that examined a similar question in the context of arithmetic. Church independently came to the same conclusion as Turing.29 Taken together, the works of Turing, Church, and Gödel were the first formal proofs that there are definite limits to what logic, mathematics, and computation can do.
In addition, Church and Turing also advanced, independently, an assertion that has become known as the Church-Turing thesis. This thesis has both weak and strong interpretations. The weak interpretation is that if a problem that can be presented to a Turing machine is not solvable by one, then it is not solvable by any machine. This conclusion follows from Turing’s demonstration that the Turing machine could model any algorithmic process. It is only a small step from there to describe the behavior of a machine as following an algorithm.
The strong interpretation is that problems that are not solvable on a Turing machine cannot be solved by human thought, either. The basis of this thesis is that human thought is performed by the human brain (with some influence by the body), that the human brain (and body) comprises matter and energy, that matter and energy follow natural laws, that these laws are describable in mathematical terms, and that mathematics can be simulated to any degree of precision by algorithms. Therefore there exist algorithms that can simulate human thought. The strong version of the Church-Turing thesis postulates an essential equivalence between what a human can think or know and what is computable.
It is important to note that although the existence of Turing’s unsolvable problems is a mathematical certainty, the Church-Turing thesis is not a mathematical proposition at all. It is, rather, a conjecture that, in various disguises, is at the heart of some of our most profound debates in the philosophy of mind.30
The criticism of strong AI based on the Church-Turing thesis argues the following: since there are clear limitations to the types of problems that a computer can solve, yet humans are capable of solving these problems, machines will never emulate the full range of human intelligence. This conclusion, however, is not warranted. Humans are no more capable of universally solving such “unsolvable” problems than machines are. We can make educated guesses to solutions in certain instances and can apply heuristic methods (procedures that attempt to solve problems but that are not guaranteed to work) that succeed on occasion. But both these approaches are also algorithmically based processes, which means that machines are also capable of doing them. Indeed, machines can often search for solutions with far greater speed and thoroughness than humans can.
The strong formulation of the Church-Turing thesis implies that biological brains and machines are equally subject to the laws of physics, and therefore mathematics can model and simulate them equally. We’ve already demonstrated the ability to model and simulate the function of neurons, so why not a system of a hundred billion neurons? Such a system would display the same complexity and lack of predictability as human intelligence. Indeed, we already have computer algorithms (for example, genetic algorithms) with results that are complex and unpredictable and that provide intelligent solutions to problems. If anything, the Church-Turing thesis implies that brains and machines are essentially equivalent.
To see machines’ ability to use heuristic methods, consider one of the most interesting of the unsolvable problems, the “busy beaver” problem, formulated by Tibor Rado in 1962.31 Each Turing machine has a certain number of states that its internal program can be in, which correspond to the number of steps in its internal program. There are a number of different 4-state Turing machines that are possible, a certain number of 5-state machines, and so on. In the “busy beaver” problem, given a positive integer n, we construct all the Turing machines that have n states. The number of such machines will always be finite. Next we eliminate those n-state machines that get into an infinite loop (that is, never halt). Finally, we select the machine (one that does halt) that writes the largest number of 1s on its tape. The number of 1s that this Turing machine writes is called the busy beaver of n. Rado showed that there is no algorithm—that is, no Turing machine—that can compute this function for all ns. The crux of the problem is sorting out those n-state machines that get into infinite loops. If we program a Turing machine to generate and simulate all possible n-state Turing machines, this simulator itself gets into an infinite loop when it attempts to simulate one of the n-state machines that gets into an infinite loop.
Despite its status as an unsolvable problem (and one of the most famous), we can determine the busy-beaver function for some ns. (Interestingly, it is also an unsolvable problem to separate those ns for which we can determine the busy beaver of n from those for which we cannot.) For example, the busy beaver of 6 is easily determined to be 35. With seven states, a Turing machine can multiply, so the busy beaver of 7 is much bigger: 22,961. With eight states, a Turing machine can compute exponentials, so the busy beaver of 8 is even bigger: approximately 1043. We can see that this is an “intelligent” function, in that it requires greater intelligence to solve for larger ns.
By the time we get to 10, a Turing machine can perform types of calculations that are impossible for a human to follow (without help from a computer). So we were able to determine the busy beaver of 10 only with a computer’s assistance. The answer requires an exotic notation to write down, in which we have a stack of exponents, the height of which is determined by another stack of exponents, the height of which is determined by another stack of exponents, and so on. Because a computer can keep track of such complex numbers, whereas the human brain cannot, it appears that computers will prove more capable of solving unsolvable problems than humans will.
The Criticism from Failure Rates
Jaron Lanier, Thomas Ray, and other observers all cite high failure rates of technology as a barrier to its continued exponential growth. For example, Ray writes:
The most complex of our creations are showing alarming failure rates. Orbiting satellites and telescopes, space shuttles, interplanetary probes, the Pentium chip, computer operating systems, all seem to be pushing the limits of what we can effectively design and build through conventional approaches….Our most complex software (operating systems and telecommunications control systems) already contains tens of millions of lines of code. At present it seems unlikely that we can produce and manage software with hundreds of millions or billions of lines of code.32
First, we might ask what alarming failure rates Ray is referring to. As mentioned earlier, computerized systems of significant sophistication routinely fly and land our airplanes automatically and monitor intensive care units in hospitals, yet almost never malfunction. If alarming failure rates are of concern, they’re more often attributable to human error. Ray alludes to problems with Intel microprocessor chips, but these problems have been extremely subtle, have caused almost no repercussions, and have quickly been rectified.
The complexity of computerized systems has indeed been scaling up, as we have seen, and moreover the cutting edge of our efforts to emulate human intelligence will utilize the self-organizing paradigms that we find in the human brain. As we continue our progress in reverse engineering the human brain, we will add new self-organizing methods to our pattern recognition and AI toolkit. As I have discussed, self-organizing methods help to alleviate the need for unmanageable levels of complexity. As I pointed out earlier, we will not need systems with “billions of lines of code” to emulate human intelligence.
It is also important to point out that imperfection is an inherent feature of any complex process, and that certainly includes human intelligence.
The Criticism from “Lock-In”
[Not shown]
The Criticism from Ontology: Can a Computer Be Conscious?
Because we do not understand the brain very well we are constantly tempted to use the latest technology as a model for trying to understand it. In my childhood we were always assured that the brain was a telephone switchboard. (“What else could it be?”) I was amused to see that Sherrington, the great British neuroscientist, thought that the brain worked like a telegraph system. Freud often compared the brain to hydraulic and electromagnetic systems. Leibniz compared it to a mill, and I am told some of the ancient Greeks thought the brain functions like a catapult. At present, obviously, the metaphor is the digital computer.
—John R. Searle, “Minds, Brains, and Science”
Can a computer—a nonbiological intelligence—be conscious? We have first, of course, to agree on what the question means. As I discussed earlier, there are conflicting perspectives on what may at first appear to be a straightforward issue. Regardless of how we attempt to define the concept, however, we must acknowledge that consciousness is widely regarded as a crucial, if not essential, attribute of being human.34
John Searle, distinguished philosopher at the University of California at Berkeley, is popular among his followers for what they believe is a staunch defense of the deep mystery of human consciousness against trivialization by strong-AI “reductionists” like Ray Kurzweil. And even though I have always found Searle’s logic in his celebrated Chinese Room argument to be tautological, I had expected an elevating treatise on the paradoxes of consciousness. Thus it is with some surprise that I find Searle writing statements such as,
“human brains cause consciousness by a series of specific neurobiological processes in the brain”;
“The essential thing is to recognize that consciousness is a biological process like digestion, lactation, photosynthesis, or mitosis”;
“The brain is a machine, a biological machine to be sure, but a machine all the same. So the first step is to figure out how the brain does it and then build an artificial machine that has an equally effective mechanism for causing consciousness”; and
“We know that brains cause consciousness with specific biological mechanisms.”35
So who is being the reductionist here? Searle apparently expects that we can measure the subjectivity of another entity as readily as we measure the oxygen output of photosynthesis.
Searle writes that I “frequently cite IBM’s Deep Blue as evidence of superior intelligence in the computer.” Of course, the opposite is the case: I cite Deep Blue not to belabor the issue of chess but rather to examine the dear contrast it illustrates between the human and contemporary machine approaches to the game. As I pointed out earlier, however, the pattern-recognition ability of chess programs is increasing, so chess machines are beginning to combine the analytical strength of traditional machine intelligence with more humanlike pattern recognition. The human paradigm (of self-organizing chaotic processes) offers profound advantages: we can recognize and respond to extremely subtle patterns. But we can build machines with the same abilities. That, indeed, has been my own area of technical interest.
Searle is best known for his Chinese Room analogy and has presented various formulations of it over twenty years. One of the more complete descriptions of it appears in his 1992 book, The Rediscovery of the Mind:
I believe the best-known argument against strong AI was my Chinese room argument … that showed that a system could instantiate a program so as to give a perfect simulation of some human cognitive capacity, such as the capacity to understand Chinese, even though that system had no understanding of Chinese whatever. Simply imagine that someone who understands no Chinese is locked in a room with a lot of Chinese symbols and a computer program for answering questions in Chinese. The input to the system consists in Chinese symbols in the form of questions; the output of the system consists in Chinese symbols in answer to the questions. We might suppose that the program is so good that the answers to the questions are indistinguishable from those of a native Chinese speaker. But all the same, neither the person inside nor any other part of the system literally understands Chinese; and because the programmed computer has nothing that this system does not have, the programmed computer, qua computer, does not understand Chinese either. Because the program is purely formal or syntactical and because minds have mental or semantic contents, any attempt to produce a mind purely with computer programs leaves out the essential features of the mind.36
Searle’s descriptions illustrate a failure to evaluate the essence of either brain processes or the nonbiological processes that could replicate them. He starts with the assumption that the “man” in the room doesn’t understand anything because, after all, “he is just a computer,” thereby illuminating his own bias. Not surprisingly Searle then concludes that the computer (as implemented by the man) doesn’t understand. Searle combines this tautology with a basic contradiction: the computer doesn’t understand Chinese, yet (according to Searle) can convincingly answer questions in Chinese. But if an entity—biological or otherwise—really doesn’t understand human language, it will quickly be unmasked by a competent interlocutor. In addition, for the program to respond convincingly, it would have to be as complex as a human brain. The observers would long be dead while the man in the room spends millions of years following a program many millions of pages long.
Most important, the man is acting only as the central processing unit, a small part of a system. While the man may not see it, the understanding is distributed across the entire pattern of the program itself and the billions of notes he would have to make to follow the program. I understand English, but none of my neurons do. My understanding is represented in vast patterns of neurotransmitter strengths, synaptic clefts, and interneuronal connections. Searle fails to account for the significance of distributed patterns of information and their emergent properties.
A failure to see that computing processes are capable of being—just like the human brain—chaotic, unpredictable, messy, tentative, and emergent is behind much of the criticism of the prospect of intelligent machines that we hear from Searle and other essentially materialist philosophers. Inevitably Searle comes back to a criticism of “symbolic” computing: that orderly sequential symbolic processes cannot re-create true thinking. I think that’s correct (depending, of course, on what level we are modeling an intelligent process), but the manipulation of symbols (in the sense that Searle implies) is not the only way to build machines, or computers.
So-called computers (and part of the problem is the word “computer,” because machines can do more than “compute”) are not limited to symbolic processing. Nonbiological entities can also use the emergent self-organizing paradigm, which is a trend well under way and one that will become even more important over the next several decades. Computers do not have to use only 0 and 1, nor do they have to be all digital. Even if a computer is all digital, digital algorithms can simulate analog processes to any degree of precision (or lack of precision). Machines can be massively parallel. And machines can use chaotic emergent techniques just as the brain does.
The primary computing techniques that we have used in pattern-recognition systems do not use symbol manipulation but rather self-organizing methods such as those described in chapter 5 (neural nets, Markov models, genetic algorithms, and more complex paradigms based on brain reverse engineering). A machine that could really do what Searle describes in the Chinese Room argument would not merely be manipulating language symbols, because that approach doesn’t work. This is at the heart of the philosophical sleight of hand underlying the Chinese Room. The nature of computing is not limited to manipulating logical symbols. Something is going on in the human brain, and there is nothing that prevents these biological processes from being reverse engineered and replicated in nonbiological entities.
Adherents appear to believe that Searle’s Chinese Room argument demonstrates that machines (that is, nonbiological entities) can never truly understand anything of significance, such as Chinese. First, it is important to recognize that for this system—the person and the computer—to, as Searle puts it, “give a perfect simulation of some human cognitive capacity, such as the capacity to understand Chinese,” and to convincingly answer questions in Chinese, it must essentially pass a Chinese Turing test. Keep in mind that we are not talking about answering questions from a fixed list of stock questions (because that’s a trivial task) but answering any unanticipated question or sequence of questions from a knowledgeable human interrogator.
Now, the human in the Chinese Room has little or no significance. He is just feeding things into the computer and mechanically transmitting its output (or, alternatively, just following the rules in the program). And neither the computer nor the human needs to be in a room. Interpreting Searle’s description to imply that the man himself is implementing the program does not change anything other than to make the system far slower than real time and extremely error prone. Both the human and the room are irrelevant. The only thing that is significant is the computer (either an electronic computer or the computer comprising the man following the program).
For the computer to really perform this “perfect simulation,” it would indeed have to understand Chinese. According to the very premise it has “the capacity to understand Chinese,” so it is then entirely contradictory to say that “the programmed computer … does not understand Chinese.”
A computer and computer program as we know them today could not successfully perform the described task. So if we are to understand the computer to be like to day’s computers, then it cannot fulfill the premise. The only way that it could do so would be if it had the depth and complexity of a human. Turing’s brilliant insight in proposing his test was that convincingly answering any possible sequence of questions from an intelligent human questioner in a human language really probes all of human intelligence. A computer that is capable of accomplishing this—a computer that will exist a few decades from now—will need to be of human complexity or greater and will indeed understand Chinese in a deep way, because otherwise it would never be convincing in its claim to do so.
Merely stating, then, that the computer “does not literally understand Chinese” does not make sense, for it contradicts the entire premise of the argument. To claim that the computer is not conscious is not a compelling contention, either. To be consistent with some of Searle’s other statements, we have to conclude that we really don’t know if it is conscious or not. With regard to relatively simple machines, including to day’s computers, while we can’t state for certain that these entities are not conscious, their behavior, including their inner workings, doesn’t give us that impression. But that will not be true for a computer that can really do what is needed in the Chinese Room. Such a machine will at least seem conscious, even if we cannot say definitively whether it is or not. But just declaring that it is obvious that the computer (or the entire system of the computer, person, and room) is not conscious is far from a compelling argument.
In the quote above Searle states that “the program is purely formal or syntactical,” But as I pointed out earlier, that is a bad assumption, based on Searle’s failure to account for the requirements of such a technology. This assumption is behind much of Searle’s criticism of AI. A program that is purely formal or syntactical will not be able to understand Chinese, and it won’t “give a perfect simulation of some human cognitive capacity.”
But again, we don’t have to build our machines that way. We can build them in the same fashion that nature built the human brain: using chaotic emergent methods that are massively parallel. Furthermore, there is nothing inherent in the concept of a machine that restricts its expertise to the level of syntax alone and prevents it from mastering semantics. Indeed, if the machine inherent in Searle’s conception of the Chinese Room had not mastered semantics, it would not be able to convincingly answer questions in Chinese and thus would contradict Searle’s own premise.
In chapter 4 I discussed the ongoing effort to reverse engineer the human brain and to apply these methods to computing platforms of sufficient power. So, like a human brain, if we teach a computer Chinese, it will understand Chinese. This may seem to be an obvious statement, but it is one with which Searle takes issue. To use his own terminology, I am not talking about a simulation per se but rather a duplication of the causal powers of the massive neuron cluster that constitutes the brain, at least those causal powers salient and relevant to thinking.
Will such a copy be conscious? I don’t think the Chinese Room tells us anything about this question.
It is also important to point out that Searle’s Chinese Room argument can be applied to the human brain itself. Although it is clearly not his intent, his line of reasoning implies that the human brain has no understanding. He writes: “The computer … succeeds by manipulating formal symbols. The symbols themselves are quite meaningless: they have only the meaning we have attached to them. The computer knows nothing of this, it just shuffles the symbols.” Searle acknowledges that biological neurons are machines, so if we simply substitute the phrase “human brain” for “computer” and “neurotransmitter concentrations and related mechanisms” for “formal symbols,” we get:
The [human brain] … succeeds by manipulating [neurotransmitter concentrations and related mechanisms]. The [neurotransmitter concentrations and related mechanisms] themselves are quite meaningless: they have only the meaning we have attached to them. The [human brain] knows nothing of this, it just shuffles the [neurotransmitter concentrations and related mechanisms].
Of course, neurotransmitter concentrations and other neural details (for example, interneuronal connection and neurotransmitter patterns) have no meaning in and of themselves. The meaning and understanding that emerge in the human brain are exactly that: an emergent property of its complex patterns of activity. The same is true for machines. Although “shuffling symbols” does not have meaning in and of itself, the emergent patterns have the same potential role in nonbiological systems as they do in biological systems such as the brain. Hans Moravec has written, “Searle is looking for understanding in the wrong places….[He] seemingly cannot accept that real meaning can exist in mere patterns.37
Let’s address a second version of the Chinese Room. In this conception the room does not include a computer or a man simulating a computer but has a room full of people manipulating slips of paper with Chinese symbols on them—essentially, a lot of people simulating a computer. This system would convincingly answer questions in Chinese, but none of the participants would know Chinese, nor could we say that the whole system really knows Chinese—at least not in a conscious way. Searle then essentially ridicules the idea that this “system” could be conscious. What are we to consider conscious, he asks: the slips of paper? The room?
One of the problems with this version of the Chinese Room argument is that it does not come remotely close to really solving the specific problem of answering questions in Chinese. Instead it is really a description of a machinelike process that uses the equivalent of a table lookup, with perhaps some straightforward logical manipulations, to answer questions. It would be able to answer a limited number of canned questions, but if it were to answer any arbitrary question that it might be asked, it would really have to understand Chinese in the same way that a Chinese-speaking person does. Again, it is essentially being asked to pass a Chinese Turing test, and as such, would have to be as clever, and about as complex, as a human brain. Straightforward table lookup algorithms are simply not going to achieve that.
If we want to re-create a brain that understands Chinese using people as little cogs in the re-creation, we would really need billions of people simulating the processes in a human brain (essentially the people would be simulating a computer, which would be simulating human brain methods). This would require a rather large room, indeed. And even if extremely efficiently organized, this system would run many thousands of times slower than the Chinese-speaking brain it is attempting to re-create.
Now, it’s true that none of these billions of people would need to know anything about Chinese, and none of them would necessarily know what is going on in this elaborate system. But that’s equally true of the neural connections in a real human brain. None of the hundred trillion connections in my brain knows anything about this book I am writing, nor do any of them know English, nor any of the other things that I know. None of them is conscious of this chapter, nor of any of the things I am conscious of. Probably none of them is conscious at all. But the entire system of them—that is, Ray Kurzweil—is conscious. At least I’m claiming that I’m conscious (and so far, these claims have not been challenged).
So if we scale up Searle’s Chinese Room to be the rather massive “room” it needs to be, who’s to say that the entire system of billions of people simulating a brain that knows Chinese isn’t conscious? Certainly it would be correct to say that such a system knows Chinese. And we can’t say that it is not conscious any more than we can say that about any other brain process. We can’t know the subjective experience of another entity (and in at least some of Searle’s other writings, he appears to acknowledge this limitation). And this massive multibillion-person “room” is an entity. And perhaps it is conscious. Searle is just declaring ipso facto that it isn’t conscious and that this conclusion is obvious. It may seem that way when you call it a room and talk about a limited number of people manipulating a small number of slips of paper. But as I said, such a system doesn’t remotely work.
Another key to the philosophical confusion implicit in the Chinese Room argument is specifically related to the complexity and scale of the system. Searle says that whereas he cannot prove that his typewriter or tape recorder is not conscious, he feels it is obvious that they are not. Why is this so obvious? At least one reason is because a typewriter and a tape recorder are relatively simple entities.
But the existence or absence of consciousness is not so obvious in a system that is as complex as the human brain—indeed, one that may be a direct copy of the organization and “causal powers” of a real human brain. If such a “system” acts human and knows Chinese in a human way, is it conscious? Now the answer is no longer so obvious. What Searle is saying in the Chinese Room argument is that we take a simple “machine” and then consider how absurd it is to consider such a simple machine to be conscious. The fallacy has everything to do with the scale and complexity of the system. Complexity alone does not necessarily give us consciousness, but the Chinese Room tells us nothing about whether or not such a system is conscious.
Kurzweil’s Chinese Room. I have my own conception of the Chinese Room—call it Ray Kurzweil’s Chinese Room.
In my thought experiment there is a human in a room. The room has decorations from the Ming dynasty, including a pedestal on which sits a mechanical typewriter. The typewriter has been modified so that its keys are marked with Chinese symbols instead of English letters. And the mechanical linkages have been cleverly altered so that when the human types in a question in Chinese, the typewriter does not type the question but instead types the answer to the question. Now, the person receives questions in Chinese characters and dutifully presses the appropriate keys on the typewriter. The typewriter types out not the question, but the appropriate answer. The human then passes the answer outside the room.
So here we have a room with a human in it who appears from the outside to know Chinese yet clearly does not. And clearly the typewriter does not know Chinese, either. It is just an ordinary typewriter with its mechanical linkages modified. So despite the fact that the man in the room can answer questions in Chinese, who or what can we say truly knows Chinese? The decorations?
Now, you might have some objections to my Chinese Room.
You might point out that the decorations don’t seem to have any significance.
Yes, that’s true. Neither does the pedestal. The same can be said for the human and for the room.
You might also point out that the premise is absurd. Just changing the mechanical linkages in a mechanical typewriter could not possibly enable it to convincingly answer questions in Chinese (not to mention the fact that we can’t fit the thousands of Chinese-character symbols on the keys of a typewriter).
Yes, that’s a valid objection, as well. The only difference between my Chinese Room conception and the several proposed by Searle is that it is patently obvious in my conception that it couldn’t possibly work and is by its very nature absurd. That may not be quite as apparent to many readers or listeners with regard to the Searle Chinese Rooms. However, it is equally the case.
And yet we can make my conception work, just as we can make Searle’s conceptions work. All you have to do is to make the typewriter linkages as complex as a human brain. And that’s theoretically (if not practically) possible. But the phrase “typewriter linkages” does not suggest such vast complexity. The same is true of Searle’s description of a person manipulating slips of paper or following a book of rules or a computer program. These are all equally misleading conceptions.
Searle writes: “Actual human brains cause consciousness by a series of specific neurobiological processes in the brain.” However, he has yet to provide any basis for such a startling view. To illuminate Searle’s perspective, I quote from a letter he sent me:
It may turn out that rather simple organisms like termites or snails are conscious….The essential thing is to recognize that consciousness is a biological process like digestion, lactation, photosynthesis, or mitosis, and you should look for its specific biology as you look for the specific biology of these other processes.38
I replied:
Yes, it is true that consciousness emerges from the biological process(es) of the brain and body, but there is at least one difference. If I ask the question, “does a particular entity emit carbon dioxide,” I can answer that question through clear objective measurement. If I ask the question, “is this entity conscious,” I may be able to provide inferential arguments—possibly strong and convincing ones—but not clear objective measurement.
With regard to the snail, I wrote:
Now when you say that a snail may be conscious, I think what you are saying is the following: that we may discover a certain neurophysiological basis for consciousness (call it “x”) in humans such that when this basis was present humans were conscious, and when it was not present humans were not conscious. So we would presumably have an objectively measurable basis for consciousness. And then if we found that in a snail, we could conclude that it was conscious. But this inferential conclusion is just a strong suggestion, it is not a proof of subjective experience on the snail’s part. It may be that humans are conscious because they have “x” as well as some other quality that essentially all humans share, call this “y.” The “y” may have to do with a human’s level of complexity or something having to do with the way we are organized, or with the quantum properties of our microtubules (although this may be part of “x”), or something else entirely. The snail has “x” but doesn’t have “y” and so it may not be conscious.
How would one settle such an argument? You obviously can’t ask the snail. Even if we could imagine a way to pose the question, and it answered yes, that still wouldn’t prove that it was conscious. You can’t tell from its fairly simple and more-or-less predictable behavior. Pointing out that it has “x” may be a good argument, and many people may be convinced by it. But it’s just an argument—not a direct measurement of the snail’s subjective experience. Once again, objective measurement is incompatible with the very concept of subjective experience.
Many such arguments are taking place today—though not so much about snails as about higher-level animals. It is apparent to me that dogs and cats are conscious (and Searle has said that he acknowledges this as well). But not all humans accept this. I can imagine scientific ways of strengthening the argument by pointing out many similarities between these animals and humans, but again these are just arguments, not scientific proof.
Searle expects to find some clear biological “cause” of consciousness, and he seems unable to acknowledge that either understanding or consciousness may emerge from an overall pattern of activity. Other philosophers, such as Daniel Dennett, have articulated such “pattern emergent” theories of consciousness. But whether it is “caused” by a specific biological process or by a pattern of activity, Searle provides no foundation for how we would measure or detect consciousness. Finding a neurological correlate of consciousness in humans does not prove that consciousness is necessarily present in other entities with the same correlate, nor does it prove that the absence of such a correlate indicates the absence of consciousness. Such inferential arguments necessarily stop short of direct measurement. In this way, consciousness differs from objectively measurable processes such as lactation and photosynthesis.
As I discussed in chapter 4, we have discovered a biological feature unique to humans and a few other primates: the spindle cells. And these cells with their deep branching structures do appear to be heavily involved with our conscious responses, especially emotional ones. Is the spindle cell structure the neurophysiological basis “x” for human consciousness? What sort of experiment could possibly prove that? Cats and dogs don’t have spindle cells. Does that prove that they have no conscious experience?
Searle writes: “It is out of the question, for purely neurobiological reasons, to suppose that the chair or the computer is conscious.” I agree that chairs don’t seem to be conscious, but as for computers of the future that have the same complexity, depth, subtlety, and capabilities as humans, I don’t think we can rule out this possibility. Searle just assumes that they are not, and that it is “out of the question” to suppose otherwise. There is really nothing more of a substantive nature to Searle’s “arguments” than this tautology.
Now, part of the appeal of Searle’s stance against the possibility of a computer’s being conscious is that the computers we know today just don’t seem to be conscious. Their behavior is brittle and formulaic, even if they are occasionally unpredictable. But as I pointed out above, computers today are on the order of one million times simpler than the human brain, which is at least one reason they don’t share all of the endearing qualities of human thought. But that disparity is rapidly shrinking and will ultimately reverse itself in a couple of decades. The early twenty-first-century machines I am talking about in this book will appear and act very differently than the relatively simple computers of today.
Searle articulates the view that nonbiological entities are capable of only manipulating logical symbols and he appears to be unaware of other paradigms. It is true that manipulating symbols is largely how rule-based expert systems and game-playing programs work. But the current trend is in a different direction, toward self-organizing chaotic systems that employ biologically inspired methods, including processes derived directly from the reverse engineering of the hundreds of neuron clusters we call the human brain.
Searle acknowledges that biological neurons are machines—indeed, that the entire brain is a machine. As I discussed in chapter 4, we have already recreated in an extremely detailed way the “causal powers” of individual neurons as well as those of substantial neuron clusters. There is no conceptual barrier to scaling these efforts up to the entire human brain.
The Criticism from the Rich-Poor Divide
Another concern expressed by Jaron Lanier and others is the “terrifying” possibility that through these technologies the rich may gain certain advantages and opportunities to which the rest of humankind does not have access.39 Such inequality, of course, would be nothing new, but with regard to this issue the law of accelerating returns has an important and beneficial impact. Because of the ongoing exponential growth of price-performance, all of these technologies quickly become so inexpensive as to become almost free.
Look at the extraordinary amount of high-quality information available at no cost on the Web today that did not exist at all just a few years ago. And if one wants to point out that only a fraction of the world today has Web access, keep in mind that the explosion of the Web is still in its infancy, and access is growing exponentially. Even in the poorest countries of Africa, Web access is expanding rapidly.
Each example of information technology starts out with early-adoption versions that do not work very well and that are unaffordable except by the elite. Subsequently the technology works a bit better and becomes merely expensive. Then it works quite well and becomes inexpensive. Finally it works extremely well and is almost free. The cell phone, for example, is somewhere between these last two stages. Consider that a decade ago if a character in a movie took out a portable telephone, this was an indication that this person must be very wealthy, powerful, or both. Yet there are societies around the world in which the majority of the population were farming with their hands two decades ago and now have thriving information-based economies with widespread use of cell phones (for example, Asian societies, including rural areas of China). This lag from very expensive early adopters to very inexpensive, ubiquitous adoption now takes about a decade. But in keeping with the doubling of the paradigm-shift rate each decade, this lag will be only five years a decade from now. In twenty years, the lag will be only two to three years (see chapter 2).
The rich-poor divide remains a critical issue, and at each point in time there is more that can and should be done. It is tragic, for example, that the developed nations were not more proactive in sharing AIDS drugs with poor countries in Africa and elsewhere, with millions of lives lost as a result. But the exponential improvement in the price-performance of information technologies is rapidly mitigating this divide. Drugs are essentially an information technology, and we see the same doubling of price-performance each year as we do with other forms of information technology such as computers, communications, and DNA base-pair sequencing. AIDS drugs started out not working very well and costing tens of thousands of dollars per patient per year. Today these drugs work reasonably well and are approaching one hundred dollars per patient per year in poor countries such as those in Africa.
In chapter 2 I cited the World Bank report for 2004 of higher economic growth in the developing world (over 6 percent) compared to the world average (of 4 percent), and an overall reduction in poverty (for example, a reduction of 43 percent in extreme poverty in the East Asian and Pacific region since 1990). Moreover, economist Xavier Sala-i-Martin examined eight measures of global inequality among individuals, and found that all were declining over the past quarter century.40
The Criticism from the Likelihood of Government Regulation
These guys talking here act as though the government is not part of their lives. They may wish it weren’t, but it is. As we approach the issues they debated here today, they had better believe that those issues will be debated by the whole country. The majority of Americans will not simply sit still while some elite strips off their personalities and uploads themselves into their cyberspace paradise. They will have something to say about that. There will be vehement debate about that in this country.
—Leon Fuerth, former national security adviser to vice president Al Gore, at the 2002 Foresight Conference
Human life without death would be something other than human; consciousness of mortality gives rise to our deepest longings and greatest accomplishments.
—Leon Kass, chair of the Presidential Commission on Bioethics, 2003
The criticism concerning governmental control is that regulation will slow down and stop the acceleration of technology. Although regulation is a vital issue, it has actually had no measurable effect on the trends discussed in this book, which have occurred with extensive regulation in place. Short of a worldwide totalitarian state, the economic and other forces underlying technical progress will only grow with ongoing advances.
Consider the issue of stem-cell research, which has been especially controversial, and for which the U.S. government is restricting its funding. Stem-cell research is only one of numerous ideas concerned with controlling and influencing the information processes underlying biology that are being pursued as part of the biotechnology revolution. Even within the field of cell therapies the controversy over embryonic stem-cell research has served only to accelerate other ways of accomplishing the same goal. For example, transdifferentiation (converting one type of cell such as a skin cell into other types of cells) has moved ahead quickly.
As I reported in chapter 5, scientists have recently demonstrated the ability to reprogram skin cells into several other cell types. This approach represents the holy grail of cell therapies in that it promises an unlimited supply of differentiated cells with the patient’s own DNA. It also allows cells to be selected without DNA errors and will ultimately be able to provide extended telomere strings (to make the cells more youthful). Even embryonic stem-cell research itself has moved ahead, for example, with projects like Harvard’s major new research center and California’s successful three-billion-dollar bond initiative to support such work.
Although the restrictions on stem-cell research are unfortunate, it is hard to say that cell-therapy research, let alone the broad field of biotechnology, has been affected to a significant degree.
Some governmental restrictions reflect the perspective of fundamentalist humanism, which I addressed in the previous chapter. For example, the Council of Europe proclaimed that “human rights imply the right to inherit a genetic pattern that has not been artificially changed.”41 Perhaps the most interesting aspect of the council’s edict is its posing a restriction as a right. In the same spirit, I assume the council would advocate the human right not to be cured from natural disease by unnatural means, just as activists “protected” starving African nations from the indignity of consuming bioengineered crops.42
Ultimately the benefits of technical progress overwhelm such reflexive antitechnology sentiments. The majority of crops in the United States are already GMOs, while Asian nations are aggressively adopting the technology to feed their large populations, and even Europe is now beginning to approve GMO foods. The issue is important because unnecessary restrictions, although temporary, can result in exacerbated suffering of millions of people. But technical progress is advancing on thousands of fronts, fueled by irresistible economic gains and profound improvements in human health and well-being.
Leon Fuerth’s observation quoted above reveals an inherent misconception about information technologies. Information technologies are not available only to an elite. As discussed, desirable information technologies rapidly become ubiquitous and almost free. It is only when they don’t work very well (that is, in an early stage of development) that they are expensive and restricted to an elite.
Early in the second decade of this century, the Web will provide full immersion visual-auditory virtual reality with images written directly to our retinas from our eyeglasses and lenses and very high-bandwidth wireless Internet access woven in our clothing. These capabilities will not be restricted just to the privileged. Just like cell phones, by the time they work well they will be everywhere.
In the 2020s we will routinely have nanobots in our bloodstream keeping us healthy and augmenting our mental capabilities. By the time these work well they will be inexpensive and widely used. As I discussed above, reducing the lag between early and late adoption of information technologies will itself accelerate from the current ten-year period to only a couple of years two decades from now. Once nonbiological intelligence gets a foothold in our brains, it will at least double in capability each year, as is the nature of information technology. Thus it will not take long for the nonbiological portion of our intelligence to predominate. This will not be a luxury reserved for the rich, any more than search engines are today. And to the extent that there will be a debate about the desirability of such augmentation, it’s easy to predict who will win, since those with enhanced intelligence will be far better debaters.
The Unbearable Slowness of Social Institutions. MIT senior research scientist Joel Cutcher-Gershenfeld writes: “Just looking back over the course of the past century and a half, there have been a succession of political regimes where each was the solution to an earlier dilemma, but created new dilemmas in the subsequent era. For example, Tammany Hall and the political patron model were a vast improvement over the dominant system based on landed gentry—many more people were included in the political process. Yet, problems emerged with patronage, which led to the civil service model—a strong solution to the preceding problem by introducing the meritocracy. Then, of course, civil service became the barrier to innovation and we move to reinventing government. And the story continues.”43 Gershenfeld is pointing out that social institutions even when innovative in their day become “a drag on innovation.”
First I would point out that the conservatism of social institutions is not a new phenomenon. It is part of the evolutionary process of innovation, and the law of accelerating returns has always operated in this context. Second, innovation has a way of working around the limits imposed by institutions. The advent of decentralized technology empowers the individual to bypass all kinds of restrictions, and does represent a primary means for social change to accelerate. As one of many examples, the entire thicket of communications regulations is in the process of being bypassed by emerging point-to-point techniques such as voice over Internet protocol (VOIP).
Virtual reality will represent another means of hastening social change. People will ultimately be able to have relationships and engage in activities in immersive and highly realistic virtual-reality environments that they would not be able or willing to do in real reality.
As technology becomes more sophisticated it increasingly takes on traditional human capabilities and requires less adaptation. You had to be technically adept to use early personal computers, whereas using computerized systems today, such as cell phones, music players, and Web browsers, requires much less technical ability. In the second decade of this century, we will routinely be interacting with virtual humans that, although not yet Turing-test capable, will have sufficient natural language understanding to act as our personal assistants for a wide range of tasks.
There has always been a mix of early and late adopters of new paradigms. We still have people today who want to live as we did in the seventh century. This does not restrain the early adopters from establishing new attitudes and social conventions, for example new Web-based communities. A few hundred years ago, only a handful of people such as Leonardo da Vinci and Newton were exploring new ways of understanding and relating to the world. Today, the worldwide community that participates in and contributes to the social innovation of adopting and adapting to new technological innovation is a substantial portion of the population, another reflection of the law of accelerating returns.
The Criticism from Theism
Another common objection explicitly goes beyond science to maintain that there is a spiritual level that accounts for human capabilities and that is not penetrable by objective means. William A. Dembski, a distinguished philosopher and mathematician, decries the outlook of such thinkers as Marvin Minsky, Daniel Dennett, Patricia Churchland, and Ray Kurzweil, whom he calls “contemporary materialists” who “see the motions and modifications of matter as sufficient to account for human mentality.”44
Dembski ascribes “predictability [as] materialism’s main virtue” and cites “hollowness [as] its main fault.” He goes on to say that “humans have aspirations. We long for freedom, immortality, and the beatific vision. We are restless until we find our rest in God. The problem for the materialist, however, is that these aspirations cannot be redeemed in the coin of matter.” He concludes that humans cannot be mere machines because of “the strict absence of extra-material factors from such systems.”
I would prefer that we call Dembski’s concept of materialism “capability materialism,” or better yet “capability patternism,” Capability materialism/ pattern ism is based on the observation that biological neurons and their interconnections are made up of sustainable patterns of matter and energy. It also holds that their methods can be described, understood, and modeled with either replicas or functionally equivalent re-creations. I use the word “capability” because it encompasses all of the rich, subtle, and diverse ways in which humans interact with the world, not just those narrower skills that one might label as intellectual. Indeed, our ability to understand and respond to emotions is at least as complex and diverse as our ability to process intellectual issues.
John Searle, for example, acknowledges that human neurons are biological machines. Few serious observers have postulated capabilities or reactions of human neurons that require Dembski’s “extra-material factors.” Relying on the patterns of matter and energy in the human body and brain to explain its behavior and proficiencies need not diminish our wonderment at its remarkable qualities. Dembski has an outdated understanding of the concept of “machine.”
Dembski also writes that “unlike brains, computers are neat and precise….[C]omputers operate deterministically.” This statement and others reveal a view of machines, or entities made up of patterns of matter and energy (“material” entities), that is limited to the literally simpleminded mechanisms of nineteenth-century automatons. These devices, with their hundreds and even thousands of parts, were quite predictable and certainly not capable of longings for freedom and other such endearing qualities of the human entity. The same observations largely hold true for today’s machines, with their billions of parts. But the same cannot necessarily be said for machines with millions of billions of interacting “parts,” entities with the complexity of the human brain and body.
Moreover it is incorrect to say that materialism is predictable. Even today’s computer programs routinely use simulated randomness. If one needs truly random events in a process, there are devices that can provide this as well. Fundamentally, everything we perceive in the material world is the result of many trillions of quantum events, each of which displays a profound and irreducible quantum randomness at the core of physical reality (or so it seems—the scientific jury is still out on the true nature of the apparent randomness underlying quantum events}.The material world—at both the macro and micro levels—is anything but predictable.
Although many computer programs do operate the way Dembski describes, the predominant techniques in my own field of pattern recognition use biology-inspired chaotic-computing methods. In these systems the unpredictable interaction of millions of processes, many of which contain random and unpredictable elements, provide unexpected yet appropriate answers to subtle questions of recognition. The bulk of human intelligence consists of just these sorts of pattern-recognition processes.
As for our responses to emotions and our highest aspirations, these are properly regarded as emergent properties—profound ones to be sure but nonetheless emergent patterns that result from the interaction of the human brain with its complex environment. The complexity and capacity of nonbiological entities is increasing exponentially and will match biological systems including the human brain (along with the rest of the nervous system and the endocrine system) within a couple of decades. Indeed, many of the designs of future machines will be biologically inspired—that is, derivative of biological designs. (This is already true of many contemporary systems.) It is my thesis that by sharing the complexity as well as the actual patterns of human brains, these future nonbiological entities will display the intelligence and emotionally rich reactions (such as “aspirations”) of humans.
Will such a nonbiological entity be conscious? Searle claims that we can (at least in theory) readily resolve this question by ascertaining if it has the correct “specific neurobiological processes.” It is my view that many humans, ultimately the vast majority of humans, will come to believe that such human-derived but nonetheless nonbiological intelligent entities are conscious, but that’s a political and psychological prediction, not a scientific or philosophical judgment. My bottom line: I agree with Dembski that this is not a scientific question, because it cannot be resolved through objective observation. Some observers say that if it’s not a scientific question, it’s not an important or even a real question. My view (and I’m sure Dembski agrees) is that precisely because the question is not scientific, it is a philosophical one—indeed, the fundamental philosophical question.
Dembski writes: “We need to transcend ourselves to find ourselves. Now the motions and modifications of matter offer no opportunity for transcending ourselves….Freud … Marx … Nietzsche, … each regarded the hope for transcendence as a delusion.” This view of transcendence as an ultimate goal is reasonably stated. But I disagree that the material world offers no “opportunity for transcending.” The material world inherently evolves, and each stage transcends the stage before it. As I discussed in chapter 7, evolution moves toward greater complexity, greater elegance, greater knowledge, greater intelligence, greater beauty, greater creativity, greater love. And God has been called all these things, only without any limitation: infinite knowledge, infinite intelligence, infinite beauty, infinite creativity, and infinite love. Evolution does not achieve an infinite level, but as it explodes exponentially it certainly moves in that direction. So evolution moves inexorably toward our conception of God, albeit never reaching this ideal.
Dembski continues:
A machine is fully determined by the constitution, dynamics, and interrelationships of its physical parts “[M]achines” stresses the strict absence of extra-material factors The replacement principle is relevant to this discussion because it implies that machines have no substantive history….But a machine, properly speaking, has no history. Its history is a superfluous rider—an addendum that could easily have been different without altering the machine….For a machine, all that is is what it is at this moment. … Machines access or fail to access items in storage….Mutatis mutandis, items that represent counterfactual occurrences (i.e., things that never happened) but which are accessible can be, as far as the machine is concerned, just as though they did happen.
It need hardly be stressed that the whole point of this book is that many of our dearly held assumptions about the nature of machines and indeed of our own human nature will be called into question in the next several decades. Dembski’s conception of “history” is just another aspect of our humanity that necessarily derives from the richness, depth, and complexity of being human. Conversely, not having a history in the Dembski sense is just another attribute of the simplicity of the machines that we have known up to this time. It is precisely my thesis that machines of the 2030s and beyond will be of such great complexity and richness of organization that their behavior will evidence emotional reactions, aspirations, and, yes, history. So Dembski is merely describing today’s limited machines and just assuming that these limitations are inherent, a line of argument equivalent to stating that “today’s machines are not as capable as humans, therefore machines will never reach this level of performance.” Dembski is just assuming his conclusion.
Dembski’s view of the ability of machines to understand their own history is limited to their “accessing” items in storage. Future machines, however, will possess not only a record of their own history but an ability to understand that history and to reflect insightfully upon it. As for “items that represent counterfactual occurrences,” surely the same can be said for our human memories.
Dembski’s lengthy discussion of spirituality is summed up thus:
But how can a machine be aware of God’s presence? Recall that machines are entirely defined by the constitution, dynamics, and interrelationships among their physical parts. It follows that God cannot make his presence known to a machine by acting upon it and thereby changing its state. Indeed, the moment God acts upon a machine to change its state, it no longer properly is a machine, for an aspect of the machine now transcends its physical constituents. It follows that awareness of God’s presence by a machine must be independent of any action by God to change the state of the machine. How then does the machine come to awareness of God’s presence? The awareness must be self-induced. Machine spirituality is the spirituality of self-realization, not the spirituality of an active God who freely gives himself in self-revelation and thereby transforms the beings with which he is in communion. For Kurzweil to modify “machine” with the adjective “spiritual” therefore entails an impoverished view of spirituality.
Dembski states that an entity (for example, a person) cannot be aware of God’s presence without God’s acting upon her, yet God cannot act upon a machine, so therefore a machine cannot be aware of God’s presence. Such reasoning is entirely tautological and human-centric. God communes only with humans, and only biological ones at that. I have no problem with Dembski’s subscribing to this as a personal belief, but he fails to make the “strong case” that he promises, that “humans are not machines—period.” As with Searle, Dembski just assumes his conclusion.
Like Searle, Dembski cannot seem to grasp the concept of the emergent properties of complex distributed patterns. He writes:
Anger presumably is correlated with certain localized brain excitations. But localized brain excitations hardly explain anger any better than overt behaviors associated with anger, like shouting obscenities. Localized brain excitations may be reliably correlated with anger, but what accounts for one person interpreting a comment as an insult and experiencing anger, and another person interpreting that same comment as a joke and experiencing laughter? A full materialist account of mind needs to understand localized brain excitations in terms of other localized brain excitations. Instead we find localized brain excitations (representing, say, anger) having to be explained in terms of semantic contents (representing, say, insults). But this mixture of brain excitations and semantic contents hardly constitutes a materialist account of mind or intelligent agency.
Dembski assumes that anger is correlated with a “localized brain excitation,” but anger is almost certainly the reflection of complex distributed patterns of activity in the brain. Even if there is a localized neural correlate associated with anger, it nonetheless results from multifaceted and interacting patterns. Dembski’s question as to why different people react differently to similar situations hardly requires us to resort to his extramaterial factors for an explanation. The brains and experiences of different people are clearly not the same, and these differences are well explained by differences in their physical brains resulting from varying genes and experiences.
Dembski’s resolution of the ontological problem is that the ultimate basis of what exists is what he calls the “real world of things” that are not reducible to material stuff. Dembski does not list what “things” we might consider as fundamental, but presumably human minds would be on the list, as might be other things, such as money and chairs. There may be a small congruence of our views in this regard. I regard Dembski’s “things” as patterns. Money, for example, is a vast and persisting pattern of agreements, understandings, and expectations. “Ray Kurzweil” is perhaps not so vast a pattern but thus far is also persisting. Dembski apparently regards patterns as ephemeral and not substantial, but I have a profound respect for the power and endurance of patterns. It is not unreasonable to regard patterns as a fundamental ontological reality. We are unable to really touch matter and energy directly, but we do directly experience the patterns underlying Dembski’s “things.” Fundamental to this thesis is that as we apply our intelligence, and the extension of our intelligence called technology, to understanding the powerful patterns in our world (for example, human intelligence), we can re-create—and extend!—these patterns in other substrates. The patterns are more important than the materials that embody them.
Finally, if Dembski’s intelligence-enhancing extramaterial stuff really exists, then I’d like to know where I can get some.
The Criticism from Holism
Another common criticism says the following: machines are organized as rigidly structured hierarchies of modules, whereas biology is based on holistically-organized elements in which every element affects every other. The unique capabilities of biology (such as human intelligence) can result only from this type of holistic design. Furthermore, only biological systems can use this design principle.
Michael Denton, a biologist at the University of Otago in New Zealand, points out the apparent differences between the design principles of biological entities and those of the machines he has known. Denton eloquently describes organisms as “self-organizing, self-referential, … self-replicating, … reciprocal, … self-formative, and holistic.”45 He then makes the unsupported leap—a leap of faith, one might say—that such organic forms can be created only through biological processes and that such forms are “immutable, … impenetrable, and … fundamental” realities of existence.
I do share Denton’s “awestruck” sense of “wonderment” at the beauty, intricacy, strangeness, and interrelatedness of organic systems, ranging from the “eerie other-worldly … impression” left by asymmetric protein shapes to the extraordinary complexity of higher-order organs such as the human brain. Further, I agree with Denton that biological design represents a profound set of principles. However, it is precisely my thesis, which neither Denton nor other critics from the holistic school acknowledge or respond to, that machines (that is, entities derivative of human-directed design) can access—and already are using—these same principles. This has been the thrust of my own work and represents the wave of the future. Emulating the ideas of nature is the most effective way to harness the enormous powers that future technology will make available.
Biological systems are not completely holistic, and contemporary machines are not completely modular; both exist on a continuum. We can identify units of functionality in natural systems even at the molecular level, and discernible mechanisms of action are even more evident at the higher level of organs and brain regions. The process of understanding the functionality and information transformations performed in specific brain regions is well under way, as we discussed in chapter 4.
It is misleading to suggest that every aspect of the human brain interacts with every other aspect and that it is therefore impossible to understand its methods. Researchers have already identified and modeled the transformations of information in several dozen of its regions. Conversely there are numerous examples of contemporary machines that were not designed in a modular fashion, and in which many of the design aspects are deeply interconnected, such as the examples of genetic algorithms described in chapter 5. Denton writes:
Today almost all professional biologists have adopted the mechanistic/ reductionist approach and assume that the basic parts of an organism (like the cogs of a watch) are the primary essential things, that a living organism (like a watch) is no more than the sum of its parts, and that it is the parts that determine the properties of the whole and that (like a watch) a complete description of all the properties of an organism may be had by characterizing its parts in isolation.
Denton, too, is ignoring here the ability of complex processes to exhibit emergent properties that go beyond “its parts in isolation.” He appears to recognize this potential in nature when he writes: “In a very real sense organic forms … represent genuinely emergent realities.” However, it is hardly necessary to resort to Denton’s “vitalistic model” to explain emergent realities. Emergent properties derive from the power of patterns, and nothing restricts patterns and their emergent properties to natural systems.
Denton appears to acknowledge the feasibility of emulating the ways of nature when he writes:
Success in engineering new organic forms from proteins up to organisms will therefore require a completely novel approach, a sort of designing from “the top down.” Because the parts of organic wholes only exist in the whole, organic wholes cannot be specified bit by bit and built up from a set of relatively independent modules; consequently the entire undivided unity must be specified together in toto.
Here Denton provides sound advice and describes an approach to engineering that I and other researchers use routinely in the areas of pattern recognition, complexity (chaos) theory, and self-organizing systems. Denton appears to be unaware of these methodologies, however, and after describing examples of bottom-up, component-driven engineering and their limitations concludes with no justification that there is an unbridgeable chasm between the two design philosophies. The bridge is, in fact, already under construction.
As I discussed in chapter 5, we can create our own “eerie other-worldly” but effective designs through applied evolution. I described how to apply the principles of evolution to creating intelligent designs through genetic algorithms. In my own experience with this approach, the results are well represented by Denton’s description of organic molecules in the “apparent illogic of the design and the lack of any obvious modularity or regularity, … the sheer chaos of the arrangement, … [and the] non-mechanical impression.”
Genetic algorithms and other bottom-up self-organizing design methodologies (such as neural nets, Markov models, and others that we discussed in chapter 5) incorporate an unpredictable element, so that the results of such systems are different every time the process is run. Despite the common wisdom that machines are deterministic and therefore predictable, there are numerous readily available sources of randomness available to machines. Contemporary theories of quantum mechanics postulate a profound randomness at the core of existence. According to certain theories of quantum mechanics, what appears to be the deterministic behavior of systems at a macro level is simply the result of overwhelming statistical preponderances based on enormous numbers of fundamentally unpredictable events. Moreover, the work of Stephen Wolfram and others has demonstrated that even a system that is in theory fully deterministic can nonetheless produce effectively random and, most important, entirely unpredictable results.
Genetic algorithms and similar self-organizing approaches give rise to designs that could not have been arrived at through a modular component-driven approach. The “strangeness, … [the] chaos, … the dynamic interaction” of parts to the whole that Denton attributes exclusively to organic structures describe very well the qualities of the results of these human-initiated chaotic processes.
In my own work with genetic algorithms I have examined the process by which such an algorithm gradually improves a design. A genetic algorithm does not accomplish its design achievements through designing individual subsystems one at a time but effects an incremental “all at once” approach, making many small distributed changes throughout the design that progressively improve the overall fit or “power” of the solution. The solution itself emerges gradually and unfolds from simplicity to complexity. While the solutions it produces are often asymmetric and ungainly but effective, just as in nature, they can also appear elegant and even beautiful.
Denton is correct in observing that most contemporary machines, such as today’s conventional computers, are designed using the modular approach. There are certain significant engineering advantages to this traditional technique. For example, computers have much more accurate memories than humans and can perform logical transformations far more effectively than unaided human intelligence. Most important, computers can share their memories and patterns instantly. The chaotic nonmodular approach of nature also has clear advantages that Denton well articulates, as evidenced by the deep powers of human pattern recognition. But it is a wholly unjustified leap to say that because of the current (and diminishing!) limitations of human-directed technology that biological systems are inherently, even onto logically, a world apart.
The exquisite designs of nature (the eye, for example) have benefited from a profound evolutionary process. Our most complex genetic algorithms today incorporate genetic codes ‘of tens of thousands of bits, whereas biological entities such as humans are characterized by genetic codes of billions of bits (only tens of millions of bytes with compression).
However, as is the case with all information-based technology, the complexity of genetic algorithms and other nature-inspired methods is increasing exponentially. If we examine the rate at which this complexity is increasing, we find that they will match the complexity of human intelligence within about two decades, which is consistent with my estimates drawn from direct trends in hardware and software.
Denton points out we have not yet succeeded in folding proteins in three dimensions, “even one consisting of only 100 components.” However, it is only in the recent few years that we have had the tools even to visualize these three-dimensional patterns. Moreover, modeling the interatomic forces will require on the order of one hundred thousand billion (1014) calculations per second. In late 2004 IBM introduced a version of its Blue Gene/L supercomputer with a capability of seventy teraflops (nearly 1014 cps), which, as the name suggests, is expected to provide the ability to simulate protein folding.
We have already succeeded in cutting, splicing, and rearranging genetic codes and harnessing nature’s own biochemical factories to produce enzymes and other complex biological substances. It is true that most contemporary work of this type is done in two dimensions, but the requisite computational resources to visualize and model the far more complex three-dimensional patterns found in nature are not far from realization.
In discussions of the protein issue with Denton himself, he acknowledged that the problem would eventually be solved, estimating that it was perhaps a decade away. The fact that a certain technical feat has not yet been accomplished is not a strong argument that it never will be. Denton writes:
From knowledge of the genes of an organism it is impossible to predict the encoded organic forms. Neither the properties nor structure of individual proteins nor those of any higher order forms—such as ribosomes and whole cells—can be inferred even from the most exhaustive analysis of the genes and their primary products, linear sequences of amino acids.
Although Denton’s observation above is essentially correct, it basically points out that the genome is only part of the overall system. The DNA code is not the whole story, and the rest of the molecular support system is required for the system to work and for it to be understood. We also need the design of the ribosome and other molecules that make the DNA machinery function. However, adding these designs does not significantly change the amount of design information in biology.
But re-creating the massively parallel, digitally controlled analog, hologramlike, self-organizing, and chaotic processes of the human brain does not require us to fold proteins. As discussed in chapter 4 there are dozens of contemporary projects that have succeeded in creating detailed re-creations of neurological systems. These include neural implants that successfully function inside people’s brains without folding any proteins. However, while I understand Denton’s argument about proteins to be evidence regarding the holistic ways of nature, as I have pointed out there are no essential barriers to our emulating these ways in our technology, and we are already well down this path.
In summary, Denton is far too quick to conclude that complex systems of matter and energy in the physical world are incapable of exhibiting the “emergent … vital characteristics of organisms such as self-replication, ‘morphing,’ self-regeneration, self-assembly and the holistic order of biological design” and that, therefore, “organisms and machines belong to different categories of being.” Dembski and Denton share the same limited view of machines as entities that can be designed and constructed only in a modular way. We can build and already are building “machines” that have powers far greater than the sum of their parts by combining the self-organizing design principles of the natural world with the accelerating powers of our human-initiated technology. It will be a formidable combination.
Get The Memo
by Dr Alan D. Thompson · Be inside the lightning-fast AI revolution.Informs research at Apple, Google, Microsoft · Bestseller in 142 countries.
Artificial intelligence that matters, as it happens, in plain English.
Get The Memo.
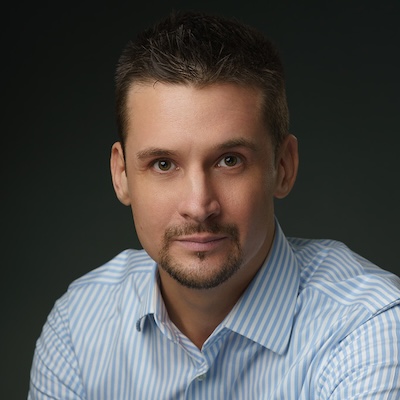
This page last updated: 30/Sep/2021. https://lifearchitect.ai/contrarianism/↑